FCN图像语义分割&ResNet50迁徙学习&ResNet50图像分类&ShuffleNet图像分类&SSD目标检测
SSD目标检测
模型简介
SSD,全称Single Shot MultiBox Detector,是Wei Liu在ECCV 2016上提出的一种目标检测算法。使用Nvidia Titan X在VOC 2007测试集上,SSD对于输入尺寸300x300的网络,达到74.3%mAP(mean Average Precision)以及59FPS;对于512x512的网络,达到了76.9%mAP ,超越当时最强的Faster RCNN(73.2%mAP)。具体可参考论文[1]。
SSD目标检测主流算法分成可以两个类型:
- two-stage方法:RCNN系列
通过算法产生候选框,然后再对这些候选框举行分类和回归。
- one-stage方法:YOLO和SSD
直接通过主干网络给出种别位置信息,不必要区域生成。
SSD是为实时目标检测而设计的。更快的R-CNN使用区域发起网络来创建边界框,并使用这些框对对象举行分类。固然它被以为是正确度的开端,但整个过程以每秒7帧的速度运行。远低于实时处置处罚所需。SSD通过消除对区域提议网络的需求来加快这一过程。为了规复正确度的下降,SSD应用了一些改进,包罗多尺度功能和默认框。这些改进使SSD可以使用更低分辨率的图像来匹配更快的R-CNN的精度,这进一步推动了速度的进步。根据下面的对比,它达到了实时的处置处罚速度,乃至超过了Faster R-CNN的精度。(正确度以平均精度mAP来衡量:预测的精度。)
SSD是单阶段的目标检测算法,通过卷积神经网络举行特征提取,取差异的特征层举行检测输出,以是SSD是一种多尺度的检测方法。在必要检测的特征层,直接使用一个3 × \times × 3卷积,举行通道的变换。SSD采用了anchor的策略,预设差异长宽比例的anchor,每一个输出特征层基于anchor预测多个检测框(4或者6)。采用了多尺度检测方法,浅层用于检测小目标,深层用于检测大目标。SSD的框架如下图:
模型布局
SSD采用VGG16作为基础模型,然后在VGG16的基础上新增了卷积层来获得更多的特征图以用于检测。SSD的网络布局如图所示。上面是SSD模型,下面是YOLO模型,可以显着看到SSD使用了多尺度的特征图做检测。
两种单阶段目标检测算法的比较:
SSD先通过卷积不断举行特征提取,在必要检测物体的网络,直接通过一个3 × \times × 3卷积得到输出,卷积的通道数由anchor数量和种别数量决定,具体为(anchor数量*(种别数量+4))。
SSD对比了YOLO系列目标检测方法,差异的是SSD通过卷积得到末了的边界框,而YOLO对末了的输出采用全连接的形式得到一维向量,对向量举行拆解得到最终的检测框。
模型特点
- 多尺度检测
在SSD的网络布局图中我们可以看到,SSD使用了多个特征层,特征层的尺寸分别是38 × \times × 38,19 × \times × 19,10 × \times × 10,5 × \times × 5,3 × \times × 3,1 × \times × 1,一共6种差异的特征图尺寸。大尺度特征图(较靠前的特征图)可以用来检测小物体,而小尺度特征图(较靠后的特征图)用来检测大物体。多尺度检测的方式,可以使得检测更加充分(SSD属于密集检测),更能检测出小目标。
- 采用卷积举行检测
与YOLO末了采用全连接层差异,SSD直接采用卷积对差异的特征图来举行提取检测结果。对于形状为m × \times × n × \times × p的特征图,只必要采用3 × \times × 3 × \times × p这样比较小的卷积核得到检测值。
- 预设anchor
在YOLOv1中,直接由网络预测目标的尺寸,这种方式使得预测框的长宽比和尺寸没有限制,难以训练。在SSD中,采用预设边界框,我们风俗称它为anchor(在SSD论文中叫default bounding boxes),预测框的尺寸在anchor的指导下举行微调。
环境准备
本案例基于MindSpore实现,开始实行前,请确保当地已经安装了mindspore、download、pycocotools、opencv-python。
- pip install -i https://pypi.mirrors.ustc.edu.cn/simple mindspore==2.2.14
- pip install -i https://pypi.mirrors.ustc.edu.cn/simple pycocotools==2.0.7
复制代码 数据准备与处置处罚
本案例所使用的数据集为COCO 2017。为了更加方便地保存和加载数据,本案例中在数据读取前首先将COCO数据集转换成MindRecord格式。使用MindSpore Record数据格式可以减少磁盘IO、网络IO开销,从而获得更好的使用体验和性能提拔。
首先我们必要下载处置处罚好的MindRecord格式的COCO数据集。
运行以下代码将数据集下载并解压到指定路径。
- from download import download
- dataset_url = "https://mindspore-website.obs.cn-north-4.myhuaweicloud.com/notebook/datasets/ssd_datasets.zip"
- path = "./"
- path = download(dataset_url, path, kind="zip", replace=True)
复制代码 输出:
- Downloading data from https://mindspore-website.obs.cn-north-4.myhuaweicloud.com/notebook/datasets/ssd_datasets.zip (16.6 MB)
- file_sizes: 100%|██████████████████████████| 17.4M/17.4M [00:00<00:00, 26.9MB/s]
- Extracting zip file...
- Successfully downloaded / unzipped to ./
复制代码 然后我们为数据处置处罚界说一些输入:
- coco_root = "./datasets/"
- anno_json = "./datasets/annotations/instances_val2017.json"
- train_cls = ['background', 'person', 'bicycle', 'car', 'motorcycle', 'airplane', 'bus',
- 'train', 'truck', 'boat', 'traffic light', 'fire hydrant',
- 'stop sign', 'parking meter', 'bench', 'bird', 'cat', 'dog',
- 'horse', 'sheep', 'cow', 'elephant', 'bear', 'zebra',
- 'giraffe', 'backpack', 'umbrella', 'handbag', 'tie',
- 'suitcase', 'frisbee', 'skis', 'snowboard', 'sports ball',
- 'kite', 'baseball bat', 'baseball glove', 'skateboard',
- 'surfboard', 'tennis racket', 'bottle', 'wine glass', 'cup',
- 'fork', 'knife', 'spoon', 'bowl', 'banana', 'apple',
- 'sandwich', 'orange', 'broccoli', 'carrot', 'hot dog', 'pizza',
- 'donut', 'cake', 'chair', 'couch', 'potted plant', 'bed',
- 'dining table', 'toilet', 'tv', 'laptop', 'mouse', 'remote',
- 'keyboard', 'cell phone', 'microwave', 'oven', 'toaster', 'sink',
- 'refrigerator', 'book', 'clock', 'vase', 'scissors',
- 'teddy bear', 'hair drier', 'toothbrush']
- train_cls_dict = {}
- for i, cls in enumerate(train_cls):
- train_cls_dict[cls] = i
复制代码 数据采样
为了使模型对于各种输入对象大小和形状更加鲁棒,SSD算法每个训练图像通过以下选项之一随机采样:
- 使用整个原始输入图像
- 采样一个区域,使采样区域和原始图片最小的交并比重叠为0.1,0.3,0.5,0.7或0.9
- 随机采样一个区域
每个采样区域的大小为原始图像大小的[0.3,1],长宽比在1/2和2之间。如果真实标签框中心在采样区域内,则保留两者重叠部分作为新图片的真实标注框。在上述采样步调之后,将每个采样区域大小调整为固定大小,并以0.5的概率水平翻转。
- import cv2
- import numpy as np
- def _rand(a=0., b=1.):
- return np.random.rand() * (b - a) + a
- def intersect(box_a, box_b):
- """Compute the intersect of two sets of boxes."""
- max_yx = np.minimum(box_a[:, 2:4], box_b[2:4])
- min_yx = np.maximum(box_a[:, :2], box_b[:2])
- inter = np.clip((max_yx - min_yx), a_min=0, a_max=np.inf)
- return inter[:, 0] * inter[:, 1]
- def jaccard_numpy(box_a, box_b):
- """Compute the jaccard overlap of two sets of boxes."""
- inter = intersect(box_a, box_b)
- area_a = ((box_a[:, 2] - box_a[:, 0]) *
- (box_a[:, 3] - box_a[:, 1]))
- area_b = ((box_b[2] - box_b[0]) *
- (box_b[3] - box_b[1]))
- union = area_a + area_b - inter
- return inter / union
- def random_sample_crop(image, boxes):
- """Crop images and boxes randomly."""
- height, width, _ = image.shape
- min_iou = np.random.choice([None, 0.1, 0.3, 0.5, 0.7, 0.9])
- if min_iou is None:
- return image, boxes
- for _ in range(50):
- image_t = image
- w = _rand(0.3, 1.0) * width
- h = _rand(0.3, 1.0) * height
- # aspect ratio constraint b/t .5 & 2
- if h / w < 0.5 or h / w > 2:
- continue
- left = _rand() * (width - w)
- top = _rand() * (height - h)
- rect = np.array([int(top), int(left), int(top + h), int(left + w)])
- overlap = jaccard_numpy(boxes, rect)
- # dropout some boxes
- drop_mask = overlap > 0
- if not drop_mask.any():
- continue
- if overlap[drop_mask].min() < min_iou and overlap[drop_mask].max() > (min_iou + 0.2):
- continue
- image_t = image_t[rect[0]:rect[2], rect[1]:rect[3], :]
- centers = (boxes[:, :2] + boxes[:, 2:4]) / 2.0
- m1 = (rect[0] < centers[:, 0]) * (rect[1] < centers[:, 1])
- m2 = (rect[2] > centers[:, 0]) * (rect[3] > centers[:, 1])
- # mask in that both m1 and m2 are true
- mask = m1 * m2 * drop_mask
- # have any valid boxes? try again if not
- if not mask.any():
- continue
- # take only matching gt boxes
- boxes_t = boxes[mask, :].copy()
- boxes_t[:, :2] = np.maximum(boxes_t[:, :2], rect[:2])
- boxes_t[:, :2] -= rect[:2]
- boxes_t[:, 2:4] = np.minimum(boxes_t[:, 2:4], rect[2:4])
- boxes_t[:, 2:4] -= rect[:2]
- return image_t, boxes_t
- return image, boxes
- def ssd_bboxes_encode(boxes):
- """Labels anchors with ground truth inputs."""
- def jaccard_with_anchors(bbox):
- """Compute jaccard score a box and the anchors."""
- # Intersection bbox and volume.
- ymin = np.maximum(y1, bbox[0])
- xmin = np.maximum(x1, bbox[1])
- ymax = np.minimum(y2, bbox[2])
- xmax = np.minimum(x2, bbox[3])
- w = np.maximum(xmax - xmin, 0.)
- h = np.maximum(ymax - ymin, 0.)
- # Volumes.
- inter_vol = h * w
- union_vol = vol_anchors + (bbox[2] - bbox[0]) * (bbox[3] - bbox[1]) - inter_vol
- jaccard = inter_vol / union_vol
- return np.squeeze(jaccard)
- pre_scores = np.zeros((8732), dtype=np.float32)
- t_boxes = np.zeros((8732, 4), dtype=np.float32)
- t_label = np.zeros((8732), dtype=np.int64)
- for bbox in boxes:
- label = int(bbox[4])
- scores = jaccard_with_anchors(bbox)
- idx = np.argmax(scores)
- scores[idx] = 2.0
- mask = (scores > matching_threshold)
- mask = mask & (scores > pre_scores)
- pre_scores = np.maximum(pre_scores, scores * mask)
- t_label = mask * label + (1 - mask) * t_label
- for i in range(4):
- t_boxes[:, i] = mask * bbox[i] + (1 - mask) * t_boxes[:, i]
- index = np.nonzero(t_label)
- # Transform to tlbr.
- bboxes = np.zeros((8732, 4), dtype=np.float32)
- bboxes[:, [0, 1]] = (t_boxes[:, [0, 1]] + t_boxes[:, [2, 3]]) / 2
- bboxes[:, [2, 3]] = t_boxes[:, [2, 3]] - t_boxes[:, [0, 1]]
- # Encode features.
- bboxes_t = bboxes[index]
- default_boxes_t = default_boxes[index]
- bboxes_t[:, :2] = (bboxes_t[:, :2] - default_boxes_t[:, :2]) / (default_boxes_t[:, 2:] * 0.1)
- tmp = np.maximum(bboxes_t[:, 2:4] / default_boxes_t[:, 2:4], 0.000001)
- bboxes_t[:, 2:4] = np.log(tmp) / 0.2
- bboxes[index] = bboxes_t
- num_match = np.array([len(np.nonzero(t_label)[0])], dtype=np.int32)
- return bboxes, t_label.astype(np.int32), num_match
- def preprocess_fn(img_id, image, box, is_training):
- """Preprocess function for dataset."""
- cv2.setNumThreads(2)
- def _infer_data(image, input_shape):
- img_h, img_w, _ = image.shape
- input_h, input_w = input_shape
- image = cv2.resize(image, (input_w, input_h))
- # When the channels of image is 1
- if len(image.shape) == 2:
- image = np.expand_dims(image, axis=-1)
- image = np.concatenate([image, image, image], axis=-1)
- return img_id, image, np.array((img_h, img_w), np.float32)
- def _data_aug(image, box, is_training, image_size=(300, 300)):
- ih, iw, _ = image.shape
- h, w = image_size
- if not is_training:
- return _infer_data(image, image_size)
- # Random crop
- box = box.astype(np.float32)
- image, box = random_sample_crop(image, box)
- ih, iw, _ = image.shape
- # Resize image
- image = cv2.resize(image, (w, h))
- # Flip image or not
- flip = _rand() < .5
- if flip:
- image = cv2.flip(image, 1, dst=None)
- # When the channels of image is 1
- if len(image.shape) == 2:
- image = np.expand_dims(image, axis=-1)
- image = np.concatenate([image, image, image], axis=-1)
- box[:, [0, 2]] = box[:, [0, 2]] / ih
- box[:, [1, 3]] = box[:, [1, 3]] / iw
- if flip:
- box[:, [1, 3]] = 1 - box[:, [3, 1]]
- box, label, num_match = ssd_bboxes_encode(box)
- return image, box, label, num_match
- return _data_aug(image, box, is_training, image_size=[300, 300])
复制代码 数据集创建
- from mindspore import Tensor
- from mindspore.dataset import MindDataset
- from mindspore.dataset.vision import Decode, HWC2CHW, Normalize, RandomColorAdjust
- def create_ssd_dataset(mindrecord_file, batch_size=32, device_num=1, rank=0,
- is_training=True, num_parallel_workers=1, use_multiprocessing=True):
- """Create SSD dataset with MindDataset."""
- dataset = MindDataset(mindrecord_file, columns_list=["img_id", "image", "annotation"], num_shards=device_num,
- shard_id=rank, num_parallel_workers=num_parallel_workers, shuffle=is_training)
- decode = Decode()
- dataset = dataset.map(operations=decode, input_columns=["image"])
- change_swap_op = HWC2CHW()
- # Computed from random subset of ImageNet training images
- normalize_op = Normalize(mean=[0.485 * 255, 0.456 * 255, 0.406 * 255],
- std=[0.229 * 255, 0.224 * 255, 0.225 * 255])
- color_adjust_op = RandomColorAdjust(brightness=0.4, contrast=0.4, saturation=0.4)
- compose_map_func = (lambda img_id, image, annotation: preprocess_fn(img_id, image, annotation, is_training))
- if is_training:
- output_columns = ["image", "box", "label", "num_match"]
- trans = [color_adjust_op, normalize_op, change_swap_op]
- else:
- output_columns = ["img_id", "image", "image_shape"]
- trans = [normalize_op, change_swap_op]
- dataset = dataset.map(operations=compose_map_func, input_columns=["img_id", "image", "annotation"],
- output_columns=output_columns, python_multiprocessing=use_multiprocessing,
- num_parallel_workers=num_parallel_workers)
- dataset = dataset.map(operations=trans, input_columns=["image"], python_multiprocessing=use_multiprocessing,
- num_parallel_workers=num_parallel_workers)
- dataset = dataset.batch(batch_size, drop_remainder=True)
- return dataset
复制代码 模型构建
SSD的网络布局主要分为以下几个部分:
- VGG16 Base Layer
- Extra Feature Layer
- Detection Layer
- NMS
- Anchor
Backbone Layer
输入图像经过预处置处罚后大小固定为300×300,首先经过backbone,本案例中使用的是VGG16网络的前13个卷积层,然后分别将VGG16的全连接层fc6和fc7转换成3 × \times × 3卷积层block6和1 × \times × 1卷积层block7,进一步提取特征。 在block6中,使用了空洞数为6的空洞卷积,其padding也为6,这样做同样也是为了增加感受野的同时保持参数量与特征图尺寸的稳定。
Extra Feature Layer
在VGG16的基础上,SSD进一步增加了4个深度卷积层,用于提取更高层的语义信息:
block8-11,用于更高语义信息的提取。block8的通道数为512,而block9、block10与block11的通道数都为256。从block7到block11,这5个卷积后输出特征图的尺寸依次为19×19、10×10、5×5、3×3和1×1。为了降低参数量,使用了1×1卷积先降低通道数为该层输出通道数的一半,再使用3×3卷积举行特征提取。
Anchor
SSD采用了PriorBox来举行区域生成。将固定大小宽高的PriorBox作为先验的感兴趣区域,使用一个阶段完成可以或许分类与回归。设计大量的密集的PriorBox保证了对整幅图像的每个地方都有检测。PriorBox位置的表现形式是以中心点坐标和框的宽、高(cx,cy,w,h)来表现的,同时都转换成百分比的形式。
PriorBox生成规则:
SSD由6个特征层来检测目标,在差异特征层上,PriorBox的尺寸scale大小是不一样的,最低层的scale=0.1,最高层的scale=0.95,其他层的盘算公式如下:
在某个特征层上其scale一定,那么会设置差异长宽比ratio的PriorBox,其长和宽的盘算公式如下:
在ratio=1的时间,还会根据该特征层和下一个特征层盘算一个特定scale的PriorBox(长宽比ratio=1),盘算公式如下:
每个特征层的每个点都会以上述规则生成PriorBox,(cx,cy)由当前点的中心点来确定,由此每个特征层都生成大量密集的PriorBox,如下图:
SSD使用了第4、7、8、9、10和11这6个卷积层得到的特征图,这6个特征图尺寸越来越小,而其对应的感受野越来越大。6个特征图上的每一个点分别对应4、6、6、6、4、4个PriorBox。某个特征图上的一个点根据下采样率可以得到在原图的坐标,以该坐标为中心生成4个或6个差异大小的PriorBox,然后使用特征图的特征去预测每一个PriorBox对应种别与位置的预丈量。比方:第8个卷积层得到的特征图大小为10×10×512,每个点对应6个PriorBox,一共有600个PriorBox。界说MultiBox类,生成多个预测框。
Detection Layer
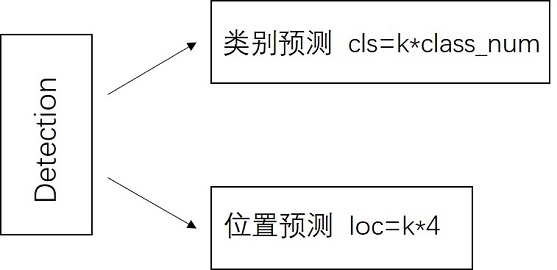
SSD模型一共有6个预测特征图,对于此中一个尺寸为m*n,通道为p的预测特征图,假设其每个像素点会产生k个anchor,每个anchor会对应c个种别和4个回归偏移量,使用(4+c)k个尺寸为3x3,通道为p的卷积查对该预测特征图举行卷积操作,得到尺寸为m*n,通道为(4+c)m*k的输出特征图,它包含了预测特征图上所产生的每个anchor的回归偏移量和各种别概率分数。以是对于尺寸为m*n的预测特征图,统共会产生(4+c)k*m*n个结果。cls分支的输出通道数为k*class_num,loc分支的输出通道数为k*4。
- from mindspore import nn
- def _make_layer(channels):
- in_channels = channels[0]
- layers = []
- for out_channels in channels[1:]:
- layers.append(nn.Conv2d(in_channels=in_channels, out_channels=out_channels, kernel_size=3))
- layers.append(nn.ReLU())
- in_channels = out_channels
- return nn.SequentialCell(layers)
- class Vgg16(nn.Cell):
- """VGG16 module."""
- def __init__(self):
- super(Vgg16, self).__init__()
- self.b1 = _make_layer([3, 64, 64])
- self.b2 = _make_layer([64, 128, 128])
- self.b3 = _make_layer([128, 256, 256, 256])
- self.b4 = _make_layer([256, 512, 512, 512])
- self.b5 = _make_layer([512, 512, 512, 512])
- self.m1 = nn.MaxPool2d(kernel_size=2, stride=2, pad_mode='SAME')
- self.m2 = nn.MaxPool2d(kernel_size=2, stride=2, pad_mode='SAME')
- self.m3 = nn.MaxPool2d(kernel_size=2, stride=2, pad_mode='SAME')
- self.m4 = nn.MaxPool2d(kernel_size=2, stride=2, pad_mode='SAME')
- self.m5 = nn.MaxPool2d(kernel_size=3, stride=1, pad_mode='SAME')
- def construct(self, x):
- # block1
- x = self.b1(x)
- x = self.m1(x)
- # block2
- x = self.b2(x)
- x = self.m2(x)
- # block3
- x = self.b3(x)
- x = self.m3(x)
- # block4
- x = self.b4(x)
- block4 = x
- x = self.m4(x)
- # block5
- x = self.b5(x)
- x = self.m5(x)
- return block4, x
复制代码- import mindspore as ms
- import mindspore.nn as nn
- import mindspore.ops as ops
- def _last_conv2d(in_channel, out_channel, kernel_size=3, stride=1, pad_mod='same', pad=0):
- in_channels = in_channel
- out_channels = in_channel
- depthwise_conv = nn.Conv2d(in_channels, out_channels, kernel_size, stride, pad_mode='same',
- padding=pad, group=in_channels)
- conv = nn.Conv2d(in_channel, out_channel, kernel_size=1, stride=1, padding=0, pad_mode='same', has_bias=True)
- bn = nn.BatchNorm2d(in_channel, eps=1e-3, momentum=0.97,
- gamma_init=1, beta_init=0, moving_mean_init=0, moving_var_init=1)
- return nn.SequentialCell([depthwise_conv, bn, nn.ReLU6(), conv])
- class FlattenConcat(nn.Cell):
- """FlattenConcat module."""
- def __init__(self):
- super(FlattenConcat, self).__init__()
- self.num_ssd_boxes = 8732
- def construct(self, inputs):
- output = ()
- batch_size = ops.shape(inputs[0])[0]
- for x in inputs:
- x = ops.transpose(x, (0, 2, 3, 1))
- output += (ops.reshape(x, (batch_size, -1)),)
- res = ops.concat(output, axis=1)
- return ops.reshape(res, (batch_size, self.num_ssd_boxes, -1))
- class MultiBox(nn.Cell):
- """
- Multibox conv layers. Each multibox layer contains class conf scores and localization predictions.
- """
- def __init__(self):
- super(MultiBox, self).__init__()
- num_classes = 81
- out_channels = [512, 1024, 512, 256, 256, 256]
- num_default = [4, 6, 6, 6, 4, 4]
- loc_layers = []
- cls_layers = []
- for k, out_channel in enumerate(out_channels):
- loc_layers += [_last_conv2d(out_channel, 4 * num_default[k],
- kernel_size=3, stride=1, pad_mod='same', pad=0)]
- cls_layers += [_last_conv2d(out_channel, num_classes * num_default[k],
- kernel_size=3, stride=1, pad_mod='same', pad=0)]
- self.multi_loc_layers = nn.CellList(loc_layers)
- self.multi_cls_layers = nn.CellList(cls_layers)
- self.flatten_concat = FlattenConcat()
- def construct(self, inputs):
- loc_outputs = ()
- cls_outputs = ()
- for i in range(len(self.multi_loc_layers)):
- loc_outputs += (self.multi_loc_layers[i](inputs[i]),)
- cls_outputs += (self.multi_cls_layers[i](inputs[i]),)
- return self.flatten_concat(loc_outputs), self.flatten_concat(cls_outputs)
- class SSD300Vgg16(nn.Cell):
- """SSD300Vgg16 module."""
- def __init__(self):
- super(SSD300Vgg16, self).__init__()
- # VGG16 backbone: block1~5
- self.backbone = Vgg16()
- # SSD blocks: block6~7
- self.b6_1 = nn.Conv2d(in_channels=512, out_channels=1024, kernel_size=3, padding=6, dilation=6, pad_mode='pad')
- self.b6_2 = nn.Dropout(p=0.5)
- self.b7_1 = nn.Conv2d(in_channels=1024, out_channels=1024, kernel_size=1)
- self.b7_2 = nn.Dropout(p=0.5)
- # Extra Feature Layers: block8~11
- self.b8_1 = nn.Conv2d(in_channels=1024, out_channels=256, kernel_size=1, padding=1, pad_mode='pad')
- self.b8_2 = nn.Conv2d(in_channels=256, out_channels=512, kernel_size=3, stride=2, pad_mode='valid')
- self.b9_1 = nn.Conv2d(in_channels=512, out_channels=128, kernel_size=1, padding=1, pad_mode='pad')
- self.b9_2 = nn.Conv2d(in_channels=128, out_channels=256, kernel_size=3, stride=2, pad_mode='valid')
- self.b10_1 = nn.Conv2d(in_channels=256, out_channels=128, kernel_size=1)
- self.b10_2 = nn.Conv2d(in_channels=128, out_channels=256, kernel_size=3, pad_mode='valid')
- self.b11_1 = nn.Conv2d(in_channels=256, out_channels=128, kernel_size=1)
- self.b11_2 = nn.Conv2d(in_channels=128, out_channels=256, kernel_size=3, pad_mode='valid')
- # boxes
- self.multi_box = MultiBox()
- def construct(self, x):
- # VGG16 backbone: block1~5
- block4, x = self.backbone(x)
- # SSD blocks: block6~7
- x = self.b6_1(x) # 1024
- x = self.b6_2(x)
- x = self.b7_1(x) # 1024
- x = self.b7_2(x)
- block7 = x
- # Extra Feature Layers: block8~11
- x = self.b8_1(x) # 256
- x = self.b8_2(x) # 512
- block8 = x
- x = self.b9_1(x) # 128
- x = self.b9_2(x) # 256
- block9 = x
- x = self.b10_1(x) # 128
- x = self.b10_2(x) # 256
- block10 = x
- x = self.b11_1(x) # 128
- x = self.b11_2(x) # 256
- block11 = x
- # boxes
- multi_feature = (block4, block7, block8, block9, block10, block11)
- pred_loc, pred_label = self.multi_box(multi_feature)
- if not self.training:
- pred_label = ops.sigmoid(pred_label)
- pred_loc = pred_loc.astype(ms.float32)
- pred_label = pred_label.astype(ms.float32)
- return pred_loc, pred_label
复制代码 损失函数
SSD算法的目标函数分为两部分:盘算相应的预选框与目标种别的置信度误差(confidence loss, conf)以及相应的位置误差(locatization loss, loc):
此中:
N 是先验框的正样本数量;
c 为种别置信度预测值;
l 为先验框的所对应边界框的位置预测值;
g 为ground truth的位置参数
α 用以调整confidence loss和location loss之间的比例,默以为1。
对于位置损失函数
针对所有的正样本,采用 Smooth L1 Loss, 位置信息都是 encode 之后的位置信息。
对于置信度损失函数
置信度损失是多类置信度©上的softmax损失。
- def class_loss(logits, label):
- """Calculate category losses."""
- label = ops.one_hot(label, ops.shape(logits)[-1], Tensor(1.0, ms.float32), Tensor(0.0, ms.float32))
- weight = ops.ones_like(logits)
- pos_weight = ops.ones_like(logits)
- sigmiod_cross_entropy = ops.binary_cross_entropy_with_logits(logits, label, weight.astype(ms.float32), pos_weight.astype(ms.float32))
- sigmoid = ops.sigmoid(logits)
- label = label.astype(ms.float32)
- p_t = label * sigmoid + (1 - label) * (1 - sigmoid)
- modulating_factor = ops.pow(1 - p_t, 2.0)
- alpha_weight_factor = label * 0.75 + (1 - label) * (1 - 0.75)
- focal_loss = modulating_factor * alpha_weight_factor * sigmiod_cross_entropy
- return focal_loss
复制代码 Metrics
在SSD中,训练过程是不必要用到非极大值克制(NMS),但当举行检测时,比方输入一张图片要求输出框的时间,必要用到NMS过滤掉那些重叠度较大的预测框。
非极大值克制的流程如下:
- 根据置信度得分举行排序
- 选择置信度最高的比边界框添加到最终输出列表中,将其从边界框列表中删除
- 盘算所有边界框的面积
- 盘算置信度最高的边界框与其它候选框的IoU
- 删除IoU大于阈值的边界框
- 重复上述过程,直至边界框列表为空
- import json
- from pycocotools.coco import COCO
- from pycocotools.cocoeval import COCOeval
- def apply_eval(eval_param_dict):
- net = eval_param_dict["net"]
- net.set_train(False)
- ds = eval_param_dict["dataset"]
- anno_json = eval_param_dict["anno_json"]
- coco_metrics = COCOMetrics(anno_json=anno_json,
- classes=train_cls,
- num_classes=81,
- max_boxes=100,
- nms_threshold=0.6,
- min_score=0.1)
- for data in ds.create_dict_iterator(output_numpy=True, num_epochs=1):
- img_id = data['img_id']
- img_np = data['image']
- image_shape = data['image_shape']
- output = net(Tensor(img_np))
- for batch_idx in range(img_np.shape[0]):
- pred_batch = {
- "boxes": output[0].asnumpy()[batch_idx],
- "box_scores": output[1].asnumpy()[batch_idx],
- "img_id": int(np.squeeze(img_id[batch_idx])),
- "image_shape": image_shape[batch_idx]
- }
- coco_metrics.update(pred_batch)
- eval_metrics = coco_metrics.get_metrics()
- return eval_metrics
- def apply_nms(all_boxes, all_scores, thres, max_boxes):
- """Apply NMS to bboxes."""
- y1 = all_boxes[:, 0]
- x1 = all_boxes[:, 1]
- y2 = all_boxes[:, 2]
- x2 = all_boxes[:, 3]
- areas = (x2 - x1 + 1) * (y2 - y1 + 1)
- order = all_scores.argsort()[::-1]
- keep = []
- while order.size > 0:
- i = order[0]
- keep.append(i)
- if len(keep) >= max_boxes:
- break
- xx1 = np.maximum(x1[i], x1[order[1:]])
- yy1 = np.maximum(y1[i], y1[order[1:]])
- xx2 = np.minimum(x2[i], x2[order[1:]])
- yy2 = np.minimum(y2[i], y2[order[1:]])
- w = np.maximum(0.0, xx2 - xx1 + 1)
- h = np.maximum(0.0, yy2 - yy1 + 1)
- inter = w * h
- ovr = inter / (areas[i] + areas[order[1:]] - inter)
- inds = np.where(ovr <= thres)[0]
- order = order[inds + 1]
- return keep
- class COCOMetrics:
- """Calculate mAP of predicted bboxes."""
- def __init__(self, anno_json, classes, num_classes, min_score, nms_threshold, max_boxes):
- self.num_classes = num_classes
- self.classes = classes
- self.min_score = min_score
- self.nms_threshold = nms_threshold
- self.max_boxes = max_boxes
- self.val_cls_dict = {i: cls for i, cls in enumerate(classes)}
- self.coco_gt = COCO(anno_json)
- cat_ids = self.coco_gt.loadCats(self.coco_gt.getCatIds())
- self.class_dict = {cat['name']: cat['id'] for cat in cat_ids}
- self.predictions = []
- self.img_ids = []
- def update(self, batch):
- pred_boxes = batch['boxes']
- box_scores = batch['box_scores']
- img_id = batch['img_id']
- h, w = batch['image_shape']
- final_boxes = []
- final_label = []
- final_score = []
- self.img_ids.append(img_id)
- for c in range(1, self.num_classes):
- class_box_scores = box_scores[:, c]
- score_mask = class_box_scores > self.min_score
- class_box_scores = class_box_scores[score_mask]
- class_boxes = pred_boxes[score_mask] * [h, w, h, w]
- if score_mask.any():
- nms_index = apply_nms(class_boxes, class_box_scores, self.nms_threshold, self.max_boxes)
- class_boxes = class_boxes[nms_index]
- class_box_scores = class_box_scores[nms_index]
- final_boxes += class_boxes.tolist()
- final_score += class_box_scores.tolist()
- final_label += [self.class_dict[self.val_cls_dict[c]]] * len(class_box_scores)
- for loc, label, score in zip(final_boxes, final_label, final_score):
- res = {}
- res['image_id'] = img_id
- res['bbox'] = [loc[1], loc[0], loc[3] - loc[1], loc[2] - loc[0]]
- res['score'] = score
- res['category_id'] = label
- self.predictions.append(res)
- def get_metrics(self):
- with open('predictions.json', 'w') as f:
- json.dump(self.predictions, f)
- coco_dt = self.coco_gt.loadRes('predictions.json')
- E = COCOeval(self.coco_gt, coco_dt, iouType='bbox')
- E.params.imgIds = self.img_ids
- E.evaluate()
- E.accumulate()
- E.summarize()
- return E.stats[0]
- class SsdInferWithDecoder(nn.Cell):
- """
- SSD Infer wrapper to decode the bbox locations."""
- def __init__(self, network, default_boxes, ckpt_path):
- super(SsdInferWithDecoder, self).__init__()
- param_dict = ms.load_checkpoint(ckpt_path)
- ms.load_param_into_net(network, param_dict)
- self.network = network
- self.default_boxes = default_boxes
- self.prior_scaling_xy = 0.1
- self.prior_scaling_wh = 0.2
- def construct(self, x):
- pred_loc, pred_label = self.network(x)
- default_bbox_xy = self.default_boxes[..., :2]
- default_bbox_wh = self.default_boxes[..., 2:]
- pred_xy = pred_loc[..., :2] * self.prior_scaling_xy * default_bbox_wh + default_bbox_xy
- pred_wh = ops.exp(pred_loc[..., 2:] * self.prior_scaling_wh) * default_bbox_wh
- pred_xy_0 = pred_xy - pred_wh / 2.0
- pred_xy_1 = pred_xy + pred_wh / 2.0
- pred_xy = ops.concat((pred_xy_0, pred_xy_1), -1)
- pred_xy = ops.maximum(pred_xy, 0)
- pred_xy = ops.minimum(pred_xy, 1)
- return pred_xy, pred_label
复制代码 训练过程
(1)先验框匹配
在训练过程中,首先要确定训练图片中的ground truth(真实目标)与哪个先验框来举行匹配,与之匹配的先验框所对应的边界框将负责预测它。
SSD的先验框与ground truth的匹配原则主要有两点:
- 对于图片中每个ground truth,找到与其IOU最大的先验框,该先验框与其匹配,这样可以保证每个ground truth一定与某个先验框匹配。通常称与ground truth匹配的先验框为正样本,反之,若一个先验框没有与任何ground truth举行匹配,那么该先验框只能与配景匹配,就是负样本。
- 对于剩余的未匹配先验框,若某个ground truth的IOU大于某个阈值(一般是0.5),那么该先验框也与这个ground truth举行匹配。尽管一个ground truth可以与多个先验框匹配,但是ground truth相对先验框还是太少了,以是负样本相对正样本会很多。为了保证正负样本只管平衡,SSD采用了hard negative mining,就是对负样本举行抽样,抽样时按照置信度误差(预测配景的置信度越小,误差越大)举行降序分列,选取误差的较大的top-k作为训练的负样本,以保证正负样本比例靠近1:3。
注意点:
- 通常称与gt匹配的prior为正样本,反之,若某一个prior没有与任何一个gt匹配,则为负样本。
- 某个gt可以和多个prior匹配,而每个prior只能和一个gt举行匹配。
- 如果多个gt和某一个prior的IOU均大于阈值,那么prior只与IOU最大的谁人举行匹配。
如上图所示,训练过程中的 prior boxes 和 ground truth boxes 的匹配,基本思路是:让每一个 prior box 回归并且到 ground truth box,这个过程的调控我们必要损失层的帮助,他管帐算真实值和预测值之间的误差,从而指导学习的走向。
(2)损失函数
损失函数使用的是上文提到的位置损失函数和置信度损失函数的加权和。
(3)数据增强
使用之前界说好的数据增强方式,对创建好的数据增强方式举行数据增强。
模型训练时,设置模型训练的epoch次数为60,然后通过create_ssd_dataset类创建了训练集和验证集。batch_size大小为5,图像尺寸同一调整为300×300。损失函数使用位置损失函数和置信度损失函数的加权和,优化器使用Momentum,并设置初始学习率为0.001。回调函数方面使用了LossMonitor和TimeMonitor来监控训练过程中每个epoch结束后,损失值Loss的厘革情况以及每个epoch、每个step的运行时间。设置每训练10个epoch保存一次模型。
- import math
- import itertools as it
- from mindspore import set_seed
- class GeneratDefaultBoxes():
- """
- Generate Default boxes for SSD, follows the order of (W, H, archor_sizes).
- `self.default_boxes` has a shape of [archor_sizes, H, W, 4], the last dimension is [y, x, h, w].
- `self.default_boxes_tlbr` has a shape as `self.default_boxes`, the last dimension is [y1, x1, y2, x2].
- """
- def __init__(self):
- fk = 300 / np.array([8, 16, 32, 64, 100, 300])
- scale_rate = (0.95 - 0.1) / (len([4, 6, 6, 6, 4, 4]) - 1)
- scales = [0.1 + scale_rate * i for i in range(len([4, 6, 6, 6, 4, 4]))] + [1.0]
- self.default_boxes = []
- for idex, feature_size in enumerate([38, 19, 10, 5, 3, 1]):
- sk1 = scales[idex]
- sk2 = scales[idex + 1]
- sk3 = math.sqrt(sk1 * sk2)
- if idex == 0 and not [[2], [2, 3], [2, 3], [2, 3], [2], [2]][idex]:
- w, h = sk1 * math.sqrt(2), sk1 / math.sqrt(2)
- all_sizes = [(0.1, 0.1), (w, h), (h, w)]
- else:
- all_sizes = [(sk1, sk1)]
- for aspect_ratio in [[2], [2, 3], [2, 3], [2, 3], [2], [2]][idex]:
- w, h = sk1 * math.sqrt(aspect_ratio), sk1 / math.sqrt(aspect_ratio)
- all_sizes.append((w, h))
- all_sizes.append((h, w))
- all_sizes.append((sk3, sk3))
- assert len(all_sizes) == [4, 6, 6, 6, 4, 4][idex]
- for i, j in it.product(range(feature_size), repeat=2):
- for w, h in all_sizes:
- cx, cy = (j + 0.5) / fk[idex], (i + 0.5) / fk[idex]
- self.default_boxes.append([cy, cx, h, w])
- def to_tlbr(cy, cx, h, w):
- return cy - h / 2, cx - w / 2, cy + h / 2, cx + w / 2
- # For IoU calculation
- self.default_boxes_tlbr = np.array(tuple(to_tlbr(*i) for i in self.default_boxes), dtype='float32')
- self.default_boxes = np.array(self.default_boxes, dtype='float32')
- default_boxes_tlbr = GeneratDefaultBoxes().default_boxes_tlbr
- default_boxes = GeneratDefaultBoxes().default_boxes
- y1, x1, y2, x2 = np.split(default_boxes_tlbr[:, :4], 4, axis=-1)
- vol_anchors = (x2 - x1) * (y2 - y1)
- matching_threshold = 0.5
复制代码- from mindspore.common.initializer import initializer, TruncatedNormal
- def init_net_param(network, initialize_mode='TruncatedNormal'):
- """Init the parameters in net."""
- params = network.trainable_params()
- for p in params:
- if 'beta' not in p.name and 'gamma' not in p.name and 'bias' not in p.name:
- if initialize_mode == 'TruncatedNormal':
- p.set_data(initializer(TruncatedNormal(0.02), p.data.shape, p.data.dtype))
- else:
- p.set_data(initialize_mode, p.data.shape, p.data.dtype)
- def get_lr(global_step, lr_init, lr_end, lr_max, warmup_epochs, total_epochs, steps_per_epoch):
- """ generate learning rate array"""
- lr_each_step = []
- total_steps = steps_per_epoch * total_epochs
- warmup_steps = steps_per_epoch * warmup_epochs
- for i in range(total_steps):
- if i < warmup_steps:
- lr = lr_init + (lr_max - lr_init) * i / warmup_steps
- else:
- lr = lr_end + (lr_max - lr_end) * (1. + math.cos(math.pi * (i - warmup_steps) / (total_steps - warmup_steps))) / 2.
- if lr < 0.0:
- lr = 0.0
- lr_each_step.append(lr)
- current_step = global_step
- lr_each_step = np.array(lr_each_step).astype(np.float32)
- learning_rate = lr_each_step[current_step:]
- return learning_rate
复制代码- import mindspore.dataset as ds
- ds.config.set_enable_shared_mem(False)
复制代码- import time
- from mindspore.amp import DynamicLossScaler
- set_seed(1)
- # load data
- mindrecord_dir = "./datasets/MindRecord_COCO"
- mindrecord_file = "./datasets/MindRecord_COCO/ssd.mindrecord0"
- dataset = create_ssd_dataset(mindrecord_file, batch_size=5, rank=0, use_multiprocessing=True)
- dataset_size = dataset.get_dataset_size()
- image, get_loc, gt_label, num_matched_boxes = next(dataset.create_tuple_iterator())
- # Network definition and initialization
- network = SSD300Vgg16()
- init_net_param(network)
- # Define the learning rate
- lr = Tensor(get_lr(global_step=0 * dataset_size,
- lr_init=0.001, lr_end=0.001 * 0.05, lr_max=0.05,
- warmup_epochs=2, total_epochs=60, steps_per_epoch=dataset_size))
- # Define the optimizer
- opt = nn.Momentum(filter(lambda x: x.requires_grad, network.get_parameters()), lr,
- 0.9, 0.00015, float(1024))
- # Define the forward procedure
- def forward_fn(x, gt_loc, gt_label, num_matched_boxes):
- pred_loc, pred_label = network(x)
- mask = ops.less(0, gt_label).astype(ms.float32)
- num_matched_boxes = ops.sum(num_matched_boxes.astype(ms.float32))
- # Positioning loss
- mask_loc = ops.tile(ops.expand_dims(mask, -1), (1, 1, 4))
- smooth_l1 = nn.SmoothL1Loss()(pred_loc, gt_loc) * mask_loc
- loss_loc = ops.sum(ops.sum(smooth_l1, -1), -1)
- # Category loss
- loss_cls = class_loss(pred_label, gt_label)
- loss_cls = ops.sum(loss_cls, (1, 2))
- return ops.sum((loss_cls + loss_loc) / num_matched_boxes)
- grad_fn = ms.value_and_grad(forward_fn, None, opt.parameters, has_aux=False)
- loss_scaler = DynamicLossScaler(1024, 2, 1000)
- # Gradient updates
- def train_step(x, gt_loc, gt_label, num_matched_boxes):
- loss, grads = grad_fn(x, gt_loc, gt_label, num_matched_boxes)
- opt(grads)
- return loss
- print("=================== Starting Training =====================")
- for epoch in range(60):
- network.set_train(True)
- begin_time = time.time()
- for step, (image, get_loc, gt_label, num_matched_boxes) in enumerate(dataset.create_tuple_iterator()):
- loss = train_step(image, get_loc, gt_label, num_matched_boxes)
- end_time = time.time()
- times = end_time - begin_time
- print(f"Epoch:[{int(epoch + 1)}/{int(60)}], "
- f"loss:{loss} , "
- f"time:{times}s ")
- ms.save_checkpoint(network, "ssd-60_9.ckpt")
- print("=================== Training Success =====================")
复制代码 评估
自界说eval_net()类对训练好的模型举行评估,调用了上述界说的SsdInferWithDecoder类返回预测的坐标及标签,然后分别盘算了在差异的IoU阈值、area和maxDets设置下的Average Precision(AP)和Average Recall(AR)。使用COCOMetrics类盘算mAP。模型在测试集上的评估指标如下。
正确率(AP)和召回率(AR)的表明
- TP:IoU>设定的阈值的检测框数量(同一Ground Truth只盘算一次)。
- FP:IoU<=设定的阈值的检测框,或者是检测到同一个GT的多余检测框的数量。
- FN:没有检测到的GT的数量。
正确率(AP)和召回率(AR)的公式
- 正确率(Average Precision,AP):
正确率是将正样本预测正确的结果与正样本预测的结果和预测错误的结果的和的比值,主要反映出预测结果错误率。
- 召回率(Average Recall,AR):
召回率是正样本预测正确的结果与正样本预测正确的结果和正样本预测错误的和的比值,主要反映出来的是预测结果中的漏检率。
关于以下代码运行结果的输出指标
- 第一个值即为mAP(mean Average Precision), 即各种别AP的平均值。
- 第二个值是iou取0.5的mAP值,是voc的评判标准。
- 第三个值是评判较为严格的mAP值,可以反应算法框的位置精准水平;中间几个数为物体大小的mAP值。
对于AR看一下maxDets=10/100的mAR值,反应检出率,如果两者靠近,分析对于这个数据集来说,不用检测出100个框,可以进步性能。
- mindrecord_file = "./datasets/MindRecord_COCO/ssd_eval.mindrecord0"
- def ssd_eval(dataset_path, ckpt_path, anno_json):
- """SSD evaluation."""
- batch_size = 1
- ds = create_ssd_dataset(dataset_path, batch_size=batch_size,
- is_training=False, use_multiprocessing=False)
- network = SSD300Vgg16()
- print("Load Checkpoint!")
- net = SsdInferWithDecoder(network, Tensor(default_boxes), ckpt_path)
- net.set_train(False)
- total = ds.get_dataset_size() * batch_size
- print("\n========================================\n")
- print("total images num: ", total)
- eval_param_dict = {"net": net, "dataset": ds, "anno_json": anno_json}
- mAP = apply_eval(eval_param_dict)
- print("\n========================================\n")
- print(f"mAP: {mAP}")
- def eval_net():
- print("Start Eval!")
- ssd_eval(mindrecord_file, "./ssd-60_9.ckpt", anno_json)
- eval_net()
复制代码 引用
[1] Liu W, Anguelov D, Erhan D, et al. Ssd: Single shot multibox detector[C]//European conference on computer vision. Springer, Cham, 2016: 21-37.
免责声明:如果侵犯了您的权益,请联系站长,我们会及时删除侵权内容,谢谢合作!更多信息从访问主页:qidao123.com:ToB企服之家,中国第一个企服评测及商务社交产业平台。 |