云计算与大数据入门实行四 —— MapReduce 低级编程实践
实行目标
- 通过实行掌握基本的 MapReduce 编程方法
- 掌握用 MapReduce 解决一些常见的数据处理问题,包罗数据去重、数据排序和数据挖掘等
实行内容
(一)编程实现文件合并和去重操作
对于两个输入文件,即文件A和文件B,请编写MapReduce步伐,对两个文件举行合并,并剔除其中重复的内容,得到一个新的输出文件C。下面是输入文件和输出文件的一个样例供参考。
输入文件A的样比方下:
- 20170101 x
- 20170102 y
- 20170103 x
- 20170104 y
- 20170105 z
- 20170106 x
复制代码 输入文件B的样比方下:
- 20170101 y
- 20170102 y
- 20170103 x
- 20170104 z
- 20170105 y
复制代码 根据输入文件A和B合并得到的输出文件C的样比方下:
- 20170101 x
- 20170101 y
- 20170102 y
- 20170103 x
- 20170104 y
- 20170104 z
- 20170105 y
- 20170105 z
- 20170106 x
复制代码 (二)编写步伐实现对输入文件的排序
如今有多个输入文件,每个文件中的每行内容均为一个整数。要求读取所有文件中的整数,举行升序排序后,输出到一个新的文件中,输出的数据格式为每行两个整数,第一个数字为第二个整数的排序位次,第二个整数为原待排列的整数。下面是输入文件和输出文件的一个样例供参考。
输入文件1的样比方下:
输入文件2的样比方下:
输入文件3的样比方下:
根据输入文件1、2和3得到的输出文件如下:
- 1 1
- 2 4
- 3 5
- 4 12
- 5 16
- 6 25
- 7 33
- 8 37
- 9 39
- 10 40
- 11 45
复制代码 (三)对给定的表格举行信息挖掘
下面给出一个child-parent的表格,要求挖掘其中的父子辈关系,给出祖孙辈关系的表格。
输入文件内容如下:
- child parent
- Steven Lucy
- Steven Jack
- Jone Lucy
- Jone Jack
- Lucy Mary
- Lucy Frank
- Jack Alice
- Jack Jesse
- David Alice
- David Jesse
- Philip David
- Philip Alma
- Mark David
- Mark Alma
复制代码 输出文件内容如下:
- grandchild grandparent
- Steven Alice
- Steven Jesse
- Jone Alice
- Jone Jesse
- Steven Mary
- Steven Frank
- Jone Mary
- Jone Frank
- Philip Alice
- Philip Jesse
- Mark Alice
- Mark Jesse
复制代码 实行步骤
对于两个输入文件,即文件 A 和文件 B,请编写 MapReduce 步伐,对两个文件举行合并,并剔除其中重复的内容,得到一个新的输出文件 C
- import java.io.IOException;
- import org.apache.hadoop.conf.Configuration;
- import org.apache.hadoop.fs.Path;
- import org.apache.hadoop.io.IntWritable;
- import org.apache.hadoop.io.Text;
- import org.apache.hadoop.mapreduce.Job;
- import org.apache.hadoop.mapreduce.Mapper;
- import org.apache.hadoop.mapreduce.Reducer;
- import org.apache.hadoop.mapreduce.lib.input.FileInputFormat;
- import org.apache.hadoop.mapreduce.lib.output.FileOutputFormat;
- import org.apache.hadoop.util.GenericOptionsParser;
- public class Main {
- /**
- // * @param args 对 A,B 两个文件进行合并,并剔除其中重复的内容,得到一个新的输出文件 C
- */
- //重载 map 函数,直接将输入中的 value 复制到输出数据的 key 上
- public static class Map extends Mapper<Object, Text, Text, Text> {
- private static Text text = new Text();
- public void map(Object key, Text value, Context context) throws
- IOException, InterruptedException {
- text = value;
- context.write(text, new Text(""));
- }
- }
- //重载 reduce 函数,直接将输入中的 key 复制到输出数据的 key 上
- public static class Reduce extends Reducer<Text, Text, Text, Text> {
- public void reduce(Text key, Iterable<Text> values, Context context)
- throws IOException, InterruptedException {
- context.write(key, new Text(""));
- }
- }
- public static void main(String[] args) throws Exception {
- // TODO Auto-generated method stub
- Configuration conf = new Configuration();
- conf.set("fs.default.name", "hdfs://localhost:8088");
- String[] otherArgs = new String[]{"input", "output"}; /* 直接设置输入参数
- */
- if (otherArgs.length != 2) {
- System.err.println("Usage: wordcount <in><out>");
- System.exit(2);
- }
- Job job = Job.getInstance(conf, "Merge and duplicate removal");
- job.setJarByClass(Main.class);
- job.setMapperClass(Map.class);
- job.setCombinerClass(Reduce.class);
- job.setReducerClass(Reduce.class);
- job.setOutputKeyClass(Text.class);
- job.setOutputValueClass(Text.class);
- FileInputFormat.addInputPath(job, new Path(otherArgs[0]));
- FileOutputFormat.setOutputPath(job, new Path(otherArgs[1]));
- System.exit(job.waitForCompletion(true) ? 0 : 1);
- }
- }
复制代码 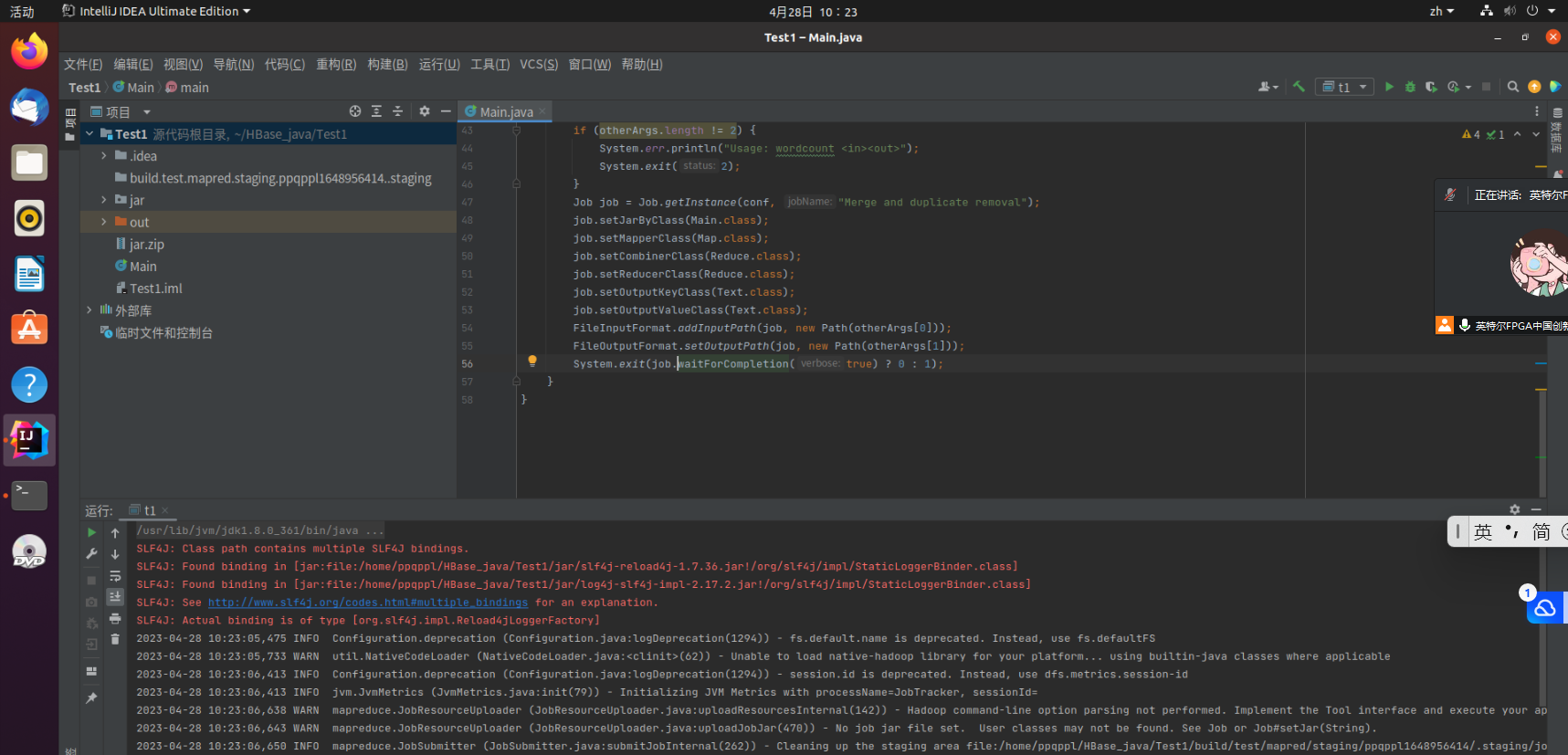
编写步伐实现对输入文件的排序
如今有多个输入文件,每个文件中的每行内容均为一个整数。要求读取所有文件中的整数,举行升序排序后,输出到一个新的文件中,输出的数据格式为每行两个整数,第一个数字为第二个整数的排序位次,第二个整数为原待排列的整数。
- import java.io.IOException;
- import org.apache.hadoop.conf.Configuration;
- import org.apache.hadoop.fs.Path;
- import org.apache.hadoop.io.IntWritable;
- import org.apache.hadoop.io.Text;
- import org.apache.hadoop.mapreduce.Job;
- import org.apache.hadoop.mapreduce.Mapper;
- import org.apache.hadoop.mapreduce.Partitioner;
- import org.apache.hadoop.mapreduce.Reducer;
- import org.apache.hadoop.mapreduce.lib.input.FileInputFormat;
- import org.apache.hadoop.mapreduce.lib.output.FileOutputFormat;
- import org.apache.hadoop.util.GenericOptionsParser;
- public class Main {
- /**
- // * @param args
- * 输入多个文件,每个文件中的每行内容均为一个整数
- * 输出到一个新的文件中,输出的数据格式为每行两个整数,第一个数字为第二个整
- 数的排序位次,第二个整数为原待排列的整数
- */
- //map 函数读取输入中的 value,将其转化成 IntWritable 类型,最后作为输出 key
- public static class Map extends Mapper<Object, Text, IntWritable, IntWritable>{
- private static IntWritable data = new IntWritable();
- public void map(Object key, Text value, Context context) throws
- IOException,InterruptedException{
- String text = value.toString();
- data.set(Integer.parseInt(text));
- context.write(data, new IntWritable(1));
- }
- }
- //reduce 函数将 map 输入的 key 复制到输出的 value 上,然后根据输入的 value-list
- // 中元素的个数决定 key 的输出次数,定义一个全局变量 line_num 来代表 key 的位次
- public static class Reduce extends Reducer<IntWritable, IntWritable,
- IntWritable, IntWritable>{
- private static IntWritable line_num = new IntWritable(1);
- public void reduce(IntWritable key, Iterable<IntWritable> values, Context
- context) throws IOException,InterruptedException{
- for(IntWritable val : values){
- context.write(line_num, key);
- line_num = new IntWritable(line_num.get() + 1);
- }
- }
- }
- //自定义 Partition 函数,此函数根据输入数据的最大值和 MapReduce 框架中
- // Partition 的数量获取将输入数据按照大小分块的边界,然后根据输入数值和边界的关系返
- // 回对应的 Partiton ID
- public static class Partition extends Partitioner<IntWritable, IntWritable>{
- public int getPartition(IntWritable key, IntWritable value, int
- num_Partition){
- int Maxnumber = 65223;//int 型的最大数值
- int bound = Maxnumber/num_Partition+1;
- int keynumber = key.get();
- for (int i = 0; i<num_Partition; i++){
- if(keynumber<bound * (i+1) && keynumber>=bound * i){
- return i;
- }
- }
- return -1;
- }
- }
- public static void main(String[] args) throws Exception{
- // TODO Auto-generated method stub
- Configuration conf = new Configuration();
- conf.set("fs.default.name","hdfs://localhost:8088");
- String[] otherArgs = new String[]{"input","output"}; /* 直接设置输入参数
- */
- if (otherArgs.length != 2) {
- System.err.println("Usage: wordcount <in><out>");
- System.exit(2);
- }
- Job job = Job.getInstance(conf,"Merge and sort");
- job.setJarByClass(Main.class);
- job.setMapperClass(Map.class);
- job.setReducerClass(Reduce.class);
- job.setPartitionerClass(Partition.class);
- job.setOutputKeyClass(IntWritable.class);
- job.setOutputValueClass(IntWritable.class);
- FileInputFormat.addInputPath(job, new Path(otherArgs[0]));
- FileOutputFormat.setOutputPath(job, new Path(otherArgs[1]));
- System.exit(job.waitForCompletion(true) ? 0 : 1);
- }
- }
复制代码 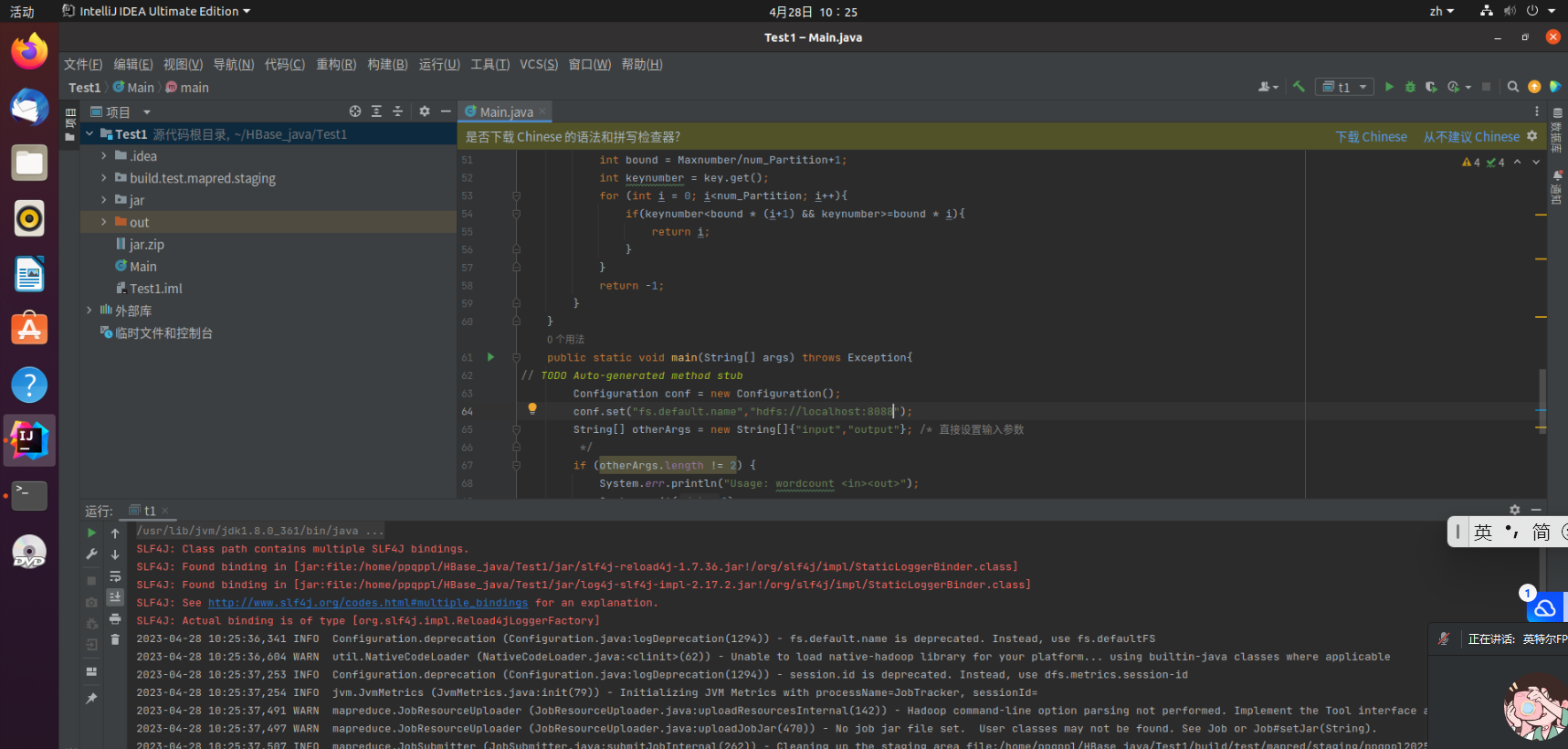
对给定的表格举行信息挖掘
下面给出一个 child-parent 的表格,要求挖掘其中的父子辈关系,给出祖孙辈关系的表格
- import java.io.IOException;
- import java.util.*;
- import org.apache.hadoop.conf.Configuration;
- import org.apache.hadoop.fs.Path;
- import org.apache.hadoop.io.IntWritable;
- import org.apache.hadoop.io.Text;
- import org.apache.hadoop.mapreduce.Job;
- import org.apache.hadoop.mapreduce.Mapper;
- import org.apache.hadoop.mapreduce.Reducer;
- import org.apache.hadoop.mapreduce.lib.input.FileInputFormat;
- import org.apache.hadoop.mapreduce.lib.output.FileOutputFormat;
- import org.apache.hadoop.util.GenericOptionsParser;
- public class Main {
- public static int time = 0;
- /**
- // * @param args
- * 输入一个 child-parent 的表格
- * 输出一个体现 grandchild-grandparent 关系的表格
- */
- //Map 将输入文件按照空格分割成 child 和 parent,然后正序输出一次作为右表,反序
- // 输出一次作为左表,
- // 需要注意的是在输出的 value
- // 中必须加上左右表区别标志
- public static class Map extends Mapper<Object, Text, Text, Text> {
- public void map(Object key, Text value, Context context) throws
- IOException, InterruptedException {
- String child_name = new String();
- String parent_name = new String();
- String relation_type = new String();
- String line = value.toString();
- int i = 0;
- while (line.charAt(i) != ' ') {
- i++;
- }
- String[] values = {line.substring(0, i), line.substring(i + 1)};
- if (values[0].compareTo("child") != 0) {
- child_name = values[0];
- parent_name = values[1];
- relation_type = "1";//左右表区分标志
- context.write(new Text(values[1]), new
- Text(relation_type + "+" + child_name + "+" + parent_name));
- //左表
- relation_type = "2";
- context.write(new Text(values[0]), new
- Text(relation_type + "+" + child_name + "+" + parent_name));
- //右表
- }
- }
- }
- public static class Reduce extends Reducer<Text, Text, Text, Text> {
- public void reduce(Text key, Iterable<Text> values, Context context) throws
- IOException, InterruptedException {
- if (time == 0) { //输出表头
- context.write(new Text("grand_child"), new
- Text("grand_parent"));
- time++;
- }
- int grand_child_num = 0;
- String grand_child[] = new String[10];
- int grand_parent_num = 0;
- String grand_parent[] = new String[10];
- Iterator ite = values.iterator();
- while (ite.hasNext()) {
- String record = ite.next().toString();
- int len = record.length();
- int i = 2;
- if (len == 0) continue;
- char relation_type = record.charAt(0);
- String child_name = new String();
- String parent_name = new String();
- //获取 value-list 中 value 的 child
- while (record.charAt(i) != '+') {
- child_name = child_name + record.charAt(i);
- i++;
- }
- i = i + 1;
- //获取 value-list 中 value 的 parent
- while (i < len) {
- parent_name = parent_name + record.charAt(i);
- i++;
- }
- //左表,取出 child 放入 grand_child
- if (relation_type == '1') {
- grand_child[grand_child_num] = child_name;
- grand_child_num++;
- } else {//右表,取出 parent 放入 grand_parent
- grand_parent[grand_parent_num] = parent_name;
- grand_parent_num++;
- }
- }
- if (grand_parent_num != 0 && grand_child_num != 0) {
- for (int m = 0; m < grand_child_num; m++) {
- for (int n = 0; n < grand_parent_num; n++) {
- context.write(new Text(grand_child[m]), new
- Text(grand_parent[n]));
- //输出结果
- }
- }
- }
- }
- }
- public static void main(String[] args) throws Exception {
- // TODO Auto-generated method stub
- Configuration conf = new Configuration();
- conf.set("fs.default.name", "hdfs://localhost:8088");
- String[] otherArgs = new String[]{"input", "output"}; /* 直接设置输入参数
- */
- if (otherArgs.length != 2) {
- System.err.println("Usage: wordcount <in><out>");
- System.exit(2);
- }
- Job job = Job.getInstance(conf, "Single table join");
- job.setJarByClass(Main.class);
- job.setMapperClass(Map.class);
- job.setReducerClass(Reduce.class);
- job.setOutputKeyClass(Text.class);
- job.setOutputValueClass(Text.class);
- FileInputFormat.addInputPath(job, new Path(otherArgs[0]));
- FileOutputFormat.setOutputPath(job, new Path(otherArgs[1]));
- System.exit(job.waitForCompletion(true) ? 0 : 1);
- }
- }
复制代码 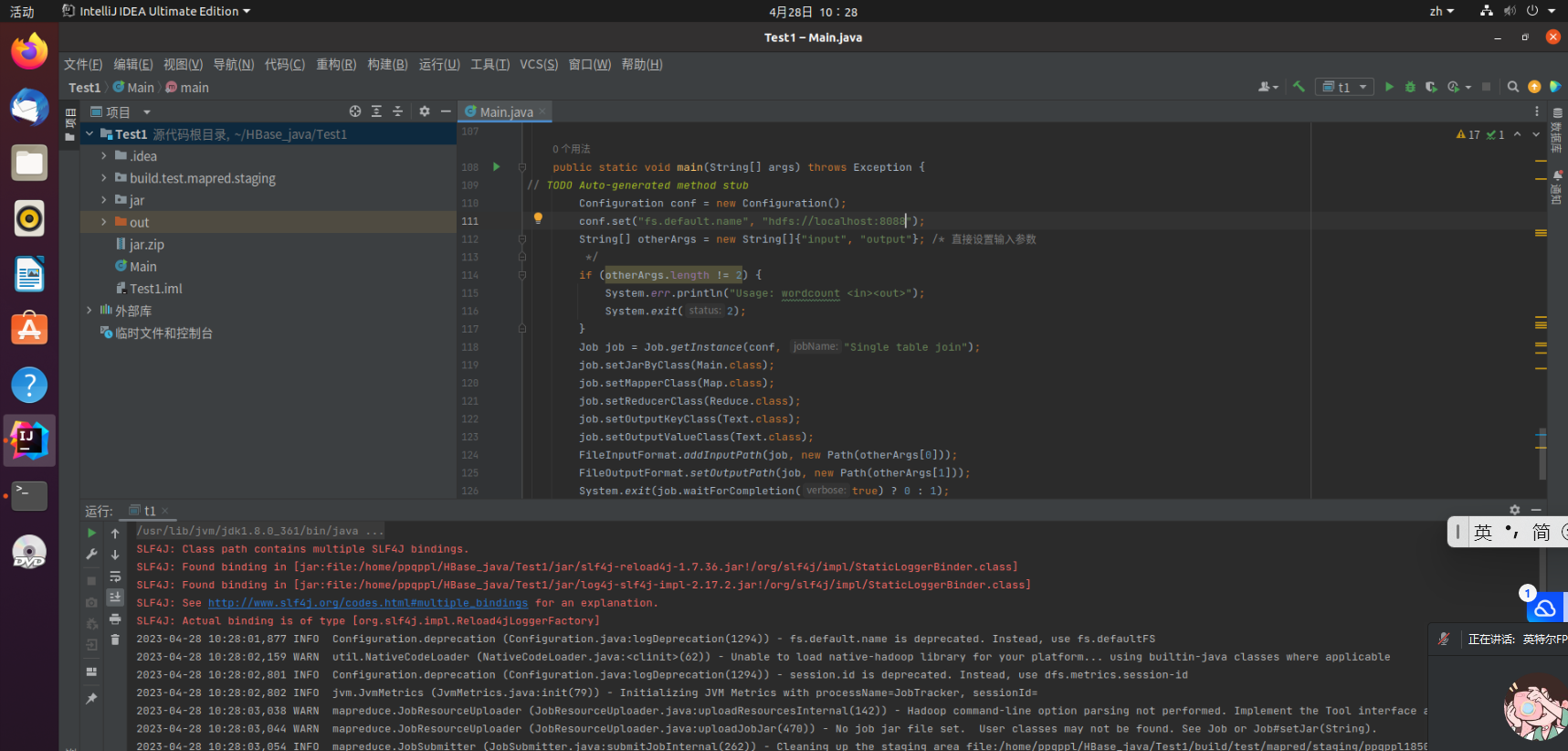
免责声明:如果侵犯了您的权益,请联系站长,我们会及时删除侵权内容,谢谢合作!更多信息从访问主页:qidao123.com:ToB企服之家,中国第一个企服评测及商务社交产业平台。 |