马上注册,结交更多好友,享用更多功能,让你轻松玩转社区。
您需要 登录 才可以下载或查看,没有账号?立即注册
x
前面先容了 android 部署大模子,下一步就是语音处置惩罚,这里我们选用 sherpa 开源项目部署语音辨认、合成、唤醒等模子。离线语音辨认库有whisper、kaldi、pocketshpinx等,在了解这些库的时间,发现了所谓“下一代Kaldi”的sherpa。从文档和模子名称看,它是一个很新的离线语音辨认库,支持中英双语辨认,文件和实时语音辨认。sherpa是一个基于下一代 Kaldi 和 onnxruntime 的开源项目,专注于语音辨认、文本转语音、说话人辨认和语音活动检测(VAD)等功能。该项目支持在没有互联网连接的情况下本地运行,实用于嵌入式系统、Android、iOS、Raspberry Pi、RISC-V 和 x86_64 服务器等多种平台。支持流式语音处置惩罚。
他有 ncnn、onnx 等平台的子项目:
https://github.com/k2-fsa/sherpa-onnx
https://github.com/k2-fsa/sherpa-ncnn
包罗的功能如下:
功能
| 形貌
| 实时语音辨认 (Streaming Speech Recognition)
| 在语音输入的同时举行处置惩罚和辨认,实用于必要即时反馈的场景,如会媾和语音助手。
| 非实时语音辨认 (Non-Streaming Speech Recognition)
| 在录制完毕后举行处置惩罚,适合必要高准确率的场景,如音频转写和文档天生。
| 文本转语音 (Text-to-Speech, TTS)
| 将文本内容转换为自然语音输出,广泛应用于语音助手和导航系统。
| 说话人分离 (Speaker Diarization)
| 辨认和区分音频流中的差别说话人,常用于集会记载和多说话人对话分析。
| 说话人辨认 (Speaker Identification)
| 确认说话者的身份,分析声纹特征并与数据库举行比对。
| 说话人验证 (Speaker Verification)
| 要求说话者提供声纹以确认身份,常用于安全性较高的场合,如银行系统。
| 口语语言辨认 (Spoken Language Identification)
| 辨认语音中使用的语言,帮助系统在多语言情况中主动切换语言。
| 音频标志 (Audio Tagging)
| 为音频内容添加标签,便于分类和搜刮,常用于音频库管理和内容推荐。
| 语音活动检测 (Voice Activity Detection, VAD)
| 检测音频流中是否存在语音活动,提拔语音辨认准确性并节流带宽和处置惩罚资源。
| 关键词检测 (Keyword Spotting)
| 辨认特定关键词或短语,常用于智能助手和语音控制装备,允许用户通过语音下令与装备交互。
| 官方参考文档:
https://k2-fsa.github.io/sherpa/onnx/index.html
1.编译
我这里使用 wsl 举行编译:
git clone https://github.com/k2-fsa/sherpa-onnx
cd sherpa-onnx
mkdir build
cd build
cmake -DCMAKE_BUILD_TYPE=Release ..
make -j6
对应的 target 如下,直接实行会有 usage说明:
add_executable(sherpa-onnx sherpa-onnx.cc)
add_executable(sherpa-onnx-keyword-spotter sherpa-onnx-keyword-spotter.cc)
add_executable(sherpa-onnx-offline sherpa-onnx-offline.cc)
add_executable(sherpa-onnx-offline-audio-tagging sherpa-onnx-offline-audio-tagging.cc)
add_executable(sherpa-onnx-offline-language-identification sherpa-onnx-offline-language-identification.cc)
add_executable(sherpa-onnx-offline-parallel sherpa-onnx-offline-parallel.cc)
add_executable(sherpa-onnx-offline-punctuation sherpa-onnx-offline-punctuation.cc)
add_executable(sherpa-onnx-online-punctuation sherpa-onnx-online-punctuation.cc)
add_executable(sherpa-onnx-offline-tts sherpa-onnx-offline-tts.cc)
add_executable(sherpa-onnx-offline-speaker-diarization sherpa-onnx-offline-speaker-diarization.cc)
add_executable(sherpa-onnx-alsa sherpa-onnx-alsa.cc alsa.cc)
add_executable(sherpa-onnx-alsa-offline sherpa-onnx-alsa-offline.cc alsa.cc)
add_executable(sherpa-onnx-alsa-offline-audio-tagging sherpa-onnx-alsa-offline-audio-tagging.cc alsa.cc)
add_executable(sherpa-onnx-alsa-offline-speaker-identification sherpa-onnx-alsa-offline-speaker-identification.cc alsa.cc)
add_executable(sherpa-onnx-keyword-spotter-alsa sherpa-onnx-keyword-spotter-alsa.cc alsa.cc)
add_executable(sherpa-onnx-vad-alsa sherpa-onnx-vad-alsa.cc alsa.cc)
add_executable(sherpa-onnx-offline-tts-play-alsa sherpa-onnx-offline-tts-play-alsa.cc alsa-play.cc)
add_executable(sherpa-onnx-offline-tts-play sherpa-onnx-offline-tts-play.cc microphone.cc)
add_executable(sherpa-onnx-keyword-spotter-microphone sherpa-onnx-keyword-spotter-microphone.cc microphone.cc)
add_executable(sherpa-onnx-microphone sherpa-onnx-microphone.cc microphone.cc)
add_executable(sherpa-onnx-microphone-offline sherpa-onnx-microphone-offline.cc microphone.cc)
add_executable(sherpa-onnx-vad-microphone sherpa-onnx-vad-microphone.cc microphone.cc)
add_executable(sherpa-onnx-vad-microphone-offline-asr sherpa-onnx-vad-microphone-offline-asr.cc microphone.cc)
add_executable(sherpa-onnx-microphone-offline-speaker-identification sherpa-onnx-microphone-offline-speaker-identification.cc microphone.cc)
add_executable(sherpa-onnx-microphone-offline-audio-tagging sherpa-onnx-microphone-offline-audio-tagging.cc microphone.cc)
add_executable(sherpa-onnx-online-websocket-server online-websocket-server-impl.cc online-websocket-server.cc)
add_executable(sherpa-onnx-online-websocket-client online-websocket-client.cc)
add_executable(sherpa-onnx-offline-websocket-server offline-websocket-server-impl.cc offline-websocket-server.cc)
说明一下他的文档上模子名称内里包罗了模子系列、语种等。
(1) 比如使用 zipformer-ctc 模子举行语音辨认:
下载模子:
cd build
wget -q https://github.com/k2-fsa/sherpa-onnx/releases/download/asr-models/sherpa-onnx-streaming-zipformer-ctc-multi-zh-hans-2023-12-13.tar.bz2
tar xvf sherpa-onnx-streaming-zipformer-ctc-multi-zh-hans-2023-12-13.tar.bz2
rm -rf sherpa-onnx-streaming-zipformer-ctc-multi-zh-hans-2023-12-13.tar.bz2
输入模子和测试wav:
./bin/sherpa-onnx \
--debug=1 \
--zipformer2-ctc-model=./sherpa-onnx-streaming-zipformer-ctc-multi-zh-hans-2023-12-13/ctc-epoch-20-avg-1-chunk-16-left-128.int8.onnx \
--tokens=./sherpa-onnx-streaming-zipformer-ctc-multi-zh-hans-2023-12-13/tokens.txt \
./sherpa-onnx-streaming-zipformer-ctc-multi-zh-hans-2023-12-13/test_wavs/DEV_T0000000000.wav
辨认结果:
./sherpa-onnx-streaming-zipformer-ctc-multi-zh-hans-2023-12-13/test_wavs/DEV_T0000000000.wav
Elapsed seconds: 1.2, Real time factor (RTF): 0.21
对我做了先容那么我想说的是各人假如对我的研究感兴趣
{ "text": " 对我做了先容那么我想说的是各人假如对我的研究感兴趣", "tokens": [" 对", "我", "做", "了", "介", "绍", "那", "么", "我", "想", "说", "的", "是", "大", "家", "如", "果", "对", "我", "的", "研", "究", "感", "兴", "趣"], "timestamps": [0.00, 0.52, 0.76, 0.84, 1.04, 1.24, 1.96, 2.04, 2.24, 2.36, 2.56, 2.68, 2.80, 3.28, 3.40, 3.60, 3.72, 3.84, 3.96, 4.04, 4.16, 4.28, 4.36, 4.60, 4.76], "ys_probs": [], "lm_probs": [], "context_scores": [], "segment": 0, "words": [], "start_time": 0.00, "is_final": false}
(2) 以及 vits-melo-tts-zh_en 模子语音合成
这个模子是他唯逐一个支持中文双语TTS的模子,带 int8 量化版本。
下载模子:
cd build
wget https://github.com/k2-fsa/sherpa-onnx/releases/download/tts-models/vits-melo-tts-zh_en.tar.bz2
tar xvf vits-melo-tts-zh_en.tar.bz2
rm vits-melo-tts-zh_en.tar.bz2
输入文本天生语音:
./bin/sherpa-onnx-offline-tts \
--vits-model=./vits-melo-tts-zh_en/model.onnx \
--vits-lexicon=./vits-melo-tts-zh_en/lexicon.txt \
--vits-tokens=./vits-melo-tts-zh_en/tokens.txt \
--vits-dict-dir=./vits-melo-tts-zh_en/dict \
--output-filename=./zh-en-0.wav \
"This is a 中英文的 text to speech 测试例子。"
2.c-api
编译动态库:
cd sherpa-onnx
mkdir build-shared
cd build-shared
cmake -DSHERPA_ONNX_ENABLE_C_API=ON -DCMAKE_BUILD_TYPE=Release -DBUILD_SHARED_LIBS=ON -DCMAKE_INSTALL_PREFIX=./ ..
make -j6
make install
编译乐成会有动态库、头文件、可实行文件路径:
bin、include、lib
下面是参考源码写的 tts、ars 测试代码:
- #include "iostream"
- #include "sherpa-onnx/c-api/c-api.h"
- #include <cstring>
- #include <stdlib.h>
- // 读取文件到内存
- static size_t ReadFile(const char *filename, const char **buffer_out) {
- FILE *file = fopen(filename, "r");
- if (file == NULL) {
- fprintf(stderr, "Failed to open %s\n", filename);
- return -1;
- }
- fseek(file, 0L, SEEK_END);
- long size = ftell(file);
- rewind(file);
- *buffer_out = static_cast<const char *>(malloc(size));
- if (*buffer_out == NULL) {
- fclose(file);
- fprintf(stderr, "Memory error\n");
- return -1;
- }
- size_t read_bytes = fread((void *)*buffer_out, 1, size, file);
- if (read_bytes != size) {
- printf("Errors occured in reading the file %s\n", filename);
- free((void *)*buffer_out);
- *buffer_out = NULL;
- fclose(file);
- return -1;
- }
- fclose(file);
- return read_bytes;
- }
- // 语音识别 asr
- void asr_1(){
- std::cout << "sherpa-onnx asr demo" << std::endl;
- // 待测试 wav
- const char *wav_filename = "/mnt/d/work/workspace/sherpa-onnx/build/sherpa-onnx-nemo-streaming-fast-conformer-transducer-en-80ms/test_wavs/0.wav";
- // 模型下载:
- // https://github.com/k2-fsa/sherpa-onnx/releases/download/asr-models/sherpa-onnx-nemo-streaming-fast-conformer-transducer-en-80ms.tar.bz2
- // Transducer 是一种基于序列到序列(seq2seq)的模型,最常用于语音识别任务中。它的流式版本支持实时处理音频输入,并输出转录结果。
- // * 架构:包含编码器(encoder)、解码器(decoder)和联合网络(joiner)。编码器将音频特征转换为隐藏向量,解码器预测输出序列,联合网络将两者结合以生成最终的输出。
- // * 应用:适合实时语音识别,尤其是在边缘设备或嵌入式设备上。
- // * 优点:支持流式解码,能够逐帧处理音频输入,具有低延迟,适用于实时语音识别应用,如语音助手、语音控制等。
- const char *tokens_path = "/mnt/d/work/workspace/sherpa-onnx/build/sherpa-onnx-nemo-streaming-fast-conformer-transducer-en-80ms/tokens.txt";
- const char *encoder_path = "/mnt/d/work/workspace/sherpa-onnx/build/sherpa-onnx-nemo-streaming-fast-conformer-transducer-en-80ms/encoder.onnx";
- const char *decoder_path = "/mnt/d/work/workspace/sherpa-onnx/build/sherpa-onnx-nemo-streaming-fast-conformer-transducer-en-80ms/decoder.onnx";
- const char *joiner_path = "/mnt/d/work/workspace/sherpa-onnx/build/sherpa-onnx-nemo-streaming-fast-conformer-transducer-en-80ms/joiner.onnx";
- // 运行参数
- const char *provider = "cpu";
- int32_t num_threads = 1;
- // 设置配置
- SherpaOnnxOnlineRecognizerConfig config = {};
- config.model_config.tokens = tokens_path; // 设定tokens路径
- config.model_config.transducer.encoder = encoder_path; // 设定encoder路径
- config.model_config.transducer.decoder = decoder_path; // 设定decoder路径
- config.model_config.transducer.joiner = joiner_path; // 设定joiner路径
- config.model_config.num_threads = num_threads; // 设置线程数
- config.model_config.provider = provider; // 使用CPU提供计算
- // 其他配置
- config.decoding_method = "greedy_search";
- config.max_active_paths = 4;
- config.feat_config.sample_rate = 16000; // 采样率
- config.feat_config.feature_dim = 80; // 输入特征 dmi
- config.enable_endpoint = 1;
- config.rule1_min_trailing_silence = 2.4;
- config.rule2_min_trailing_silence = 1.2;
- config.rule3_min_utterance_length = 300;
- // 创建 Sherpa ONNX 识别器
- const SherpaOnnxOnlineRecognizer *recognizer = SherpaOnnxCreateOnlineRecognizer(&config);
- const SherpaOnnxOnlineStream *stream = SherpaOnnxCreateOnlineStream(recognizer);
- // 模拟加载音频文件并进行解码
- const SherpaOnnxWave *wave = SherpaOnnxReadWave(wav_filename);
- if (wave == nullptr) {
- std::cerr << "Failed to read " << wav_filename << std::endl;
- return;
- }
- // 模拟流式解码
- int32_t N = 3200; // 每次处理3200个样本
- int32_t k = 0;
- while (k < wave->num_samples) {
- int32_t start = k;
- int32_t end = (start + N > wave->num_samples) ? wave->num_samples : (start + N);
- k += N;
- // 处理音频流
- SherpaOnnxOnlineStreamAcceptWaveform(stream, wave->sample_rate, wave->samples + start, end - start);
- while (SherpaOnnxIsOnlineStreamReady(recognizer, stream)) {
- SherpaOnnxDecodeOnlineStream(recognizer, stream);
- }
- const SherpaOnnxOnlineRecognizerResult *result = SherpaOnnxGetOnlineStreamResult(recognizer, stream);
- if (strlen(result->text)) {
- std::cout << "Recognized Text: " << result->text << std::endl;
- }
- SherpaOnnxDestroyOnlineRecognizerResult(result);
- }
- // 清理资源
- SherpaOnnxFreeWave(wave);
- SherpaOnnxDestroyOnlineStream(stream);
- SherpaOnnxDestroyOnlineRecognizer(recognizer);
- std::cout << "Sherpa-ONNX Test Completed" << std::endl;
- }
- // 语音识别 asr
- void asr_2(){
- // 模型下载:
- // 模型 Streaming zipformer2 CTC 的使用可以参考源码 streaming-ctc-buffered-tokens-c-api.c
- // https://github.com/k2-fsa/sherpa-onnx/releases/download/asr-models/sherpa-onnx-streaming-zipformer-ctc-multi-zh-hans-2023-12-13.tar.bz2
- // Zipformer 是一种高效的模型架构,结合了压缩和时序信息提取技术。其流式版本采用 CTC (Connectionist Temporal Classification) 作为解码方法。
- // * CTC 解码:是一种不依赖于精确对齐的解码算法,适合用于长度不匹配的输入和输出序列之间的预测,如语音识别中的不规则发音长度。
- // * Zipformer2 的特点在于其模型能够在保持较低计算成本的同时提供高准确率。
- // * 应用:支持中文多方言、跨语言的实时语音识别,尤其适用于处理大批量输入音频。
- const char *wav_filename = "/mnt/d/work/workspace/sherpa-onnx/build/sherpa-onnx-streaming-zipformer-ctc-multi-zh-hans-2023-12-13/test_wavs/DEV_T0000000000.wav";
- const char *model_filename = "/mnt/d/work/workspace/sherpa-onnx/build/sherpa-onnx-streaming-zipformer-ctc-multi-zh-hans-2023-12-13/ctc-epoch-20-avg-1-chunk-16-left-128.int8.onnx";
- const char *tokens_filename = "/mnt/d/work/workspace/sherpa-onnx/build/sherpa-onnx-streaming-zipformer-ctc-multi-zh-hans-2023-12-13/tokens.txt";
- const char *provider = "cpu";
- // Streaming zipformer2 CTC 配置
- SherpaOnnxOnlineZipformer2CtcModelConfig zipformer2_ctc_config;
- memset(&zipformer2_ctc_config, 0, sizeof(zipformer2_ctc_config));
- zipformer2_ctc_config.model = model_filename;
- // 读取 tokens 到 buffers
- const char *tokens_buf;
- size_t token_buf_size = ReadFile(tokens_filename, &tokens_buf);
- if (token_buf_size < 1) {
- fprintf(stderr, "Please check your tokens.txt!\n");
- free((void *)tokens_buf);
- return;
- }
- // Online model config
- SherpaOnnxOnlineModelConfig online_model_config;
- memset(&online_model_config, 0, sizeof(online_model_config));
- online_model_config.debug = 1;
- online_model_config.num_threads = 1;
- online_model_config.provider = provider;
- online_model_config.tokens_buf = tokens_buf;
- online_model_config.tokens_buf_size = token_buf_size;
- online_model_config.zipformer2_ctc = zipformer2_ctc_config;
- // Recognizer config
- SherpaOnnxOnlineRecognizerConfig recognizer_config;
- memset(&recognizer_config, 0, sizeof(recognizer_config));
- recognizer_config.decoding_method = "greedy_search";
- recognizer_config.model_config = online_model_config;
- SherpaOnnxOnlineRecognizer *recognizer =
- SherpaOnnxCreateOnlineRecognizer(&recognizer_config);
- free((void *)tokens_buf);
- tokens_buf = NULL;
- if (recognizer == NULL) {
- fprintf(stderr, "Please check your config!\n");
- return;
- }
- const SherpaOnnxOnlineStream *stream = SherpaOnnxCreateOnlineStream(recognizer);
- // 模拟加载音频文件并进行解码
- const SherpaOnnxWave *wave = SherpaOnnxReadWave(wav_filename);
- if (wave == nullptr) {
- std::cerr << "Failed to read " << wav_filename << std::endl;
- return;
- }
- // 开始识别
- int32_t N = 3200; // 每次处理3200个样本
- int32_t k = 0;
- while (k < wave->num_samples) {
- int32_t start = k;
- int32_t end = (start + N > wave->num_samples) ? wave->num_samples : (start + N);
- k += N;
- // 处理音频流
- SherpaOnnxOnlineStreamAcceptWaveform(stream, wave->sample_rate, wave->samples + start, end - start);
- while (SherpaOnnxIsOnlineStreamReady(recognizer, stream)) {
- SherpaOnnxDecodeOnlineStream(recognizer, stream);
- }
- const SherpaOnnxOnlineRecognizerResult *result = SherpaOnnxGetOnlineStreamResult(recognizer, stream);
- if (strlen(result->text)) {
- std::cout << "Recognized Text: " << result->text << std::endl;
- }
- SherpaOnnxDestroyOnlineRecognizerResult(result);
- }
- // 清理资源
- SherpaOnnxFreeWave(wave);
- SherpaOnnxDestroyOnlineStream(stream);
- SherpaOnnxDestroyOnlineRecognizer(recognizer);
- std::cout << "Sherpa-ONNX Test Completed" << std::endl;
- };
- // 语音合成 tts
- void tts(){
- std::cout << "sherpa-onnx tts demo" << std::endl;
- // 模型下载:vits-melo-tts-zh_en
- // https://github.com/k2-fsa/sherpa-onnx/releases/download/tts-models/vits-melo-tts-zh_en.tar.bz2
- // 目前 sherpa 只有这一个同时支持中英文 tts 的模型
- const char* output_filename = "./zh-en-0.wav"; // 输出文件名
- // 模型参数
- const char *model = "/mnt/d/work/workspace/sherpa-onnx/build/vits-melo-tts-zh_en/model.onnx";
- const char *lexicon = "/mnt/d/work/workspace/sherpa-onnx/build/vits-melo-tts-zh_en/lexicon.txt";
- const char *tokens = "/mnt/d/work/workspace/sherpa-onnx/build/vits-melo-tts-zh_en/tokens.txt";
- const char *dict = "/mnt/d/work/workspace/sherpa-onnx/build/vits-melo-tts-zh_en/dict";
- // 配置模型路径及参数
- SherpaOnnxOfflineTtsConfig config;
- memset(&config, 0, sizeof(config));
- config.model.vits.model = model;
- config.model.vits.lexicon = lexicon;
- config.model.vits.tokens = tokens;
- config.model.vits.dict_dir = dict; // 字典目录
- config.model.vits.noise_scale = 0.667; // 设置噪声比例
- config.model.vits.noise_scale_w = 0.8; // 噪声权重
- config.model.vits.length_scale = 1.0; // 语速比例
- config.model.num_threads = 1; // 使用单线程
- config.model.provider = "cpu"; // 使用 CPU 作为计算设备
- config.model.debug = 0; // 不显示调试信息
- int sid = 0; // 设置 speaker ID 为 0
- const char* text = "This is a 中英文的 text to speech 测试例子。"; // 测试文本
- // 创建 TTS 对象
- SherpaOnnxOfflineTts* tts = SherpaOnnxCreateOfflineTts(&config);
- // 生成音频
- const SherpaOnnxGeneratedAudio* audio = SherpaOnnxOfflineTtsGenerate(tts, text, sid, 1.0);
- // 将生成的音频写入 wav 文件
- SherpaOnnxWriteWave(audio->samples, audio->n, audio->sample_rate, output_filename);
- // 清理生成的音频和 TTS 对象
- SherpaOnnxDestroyOfflineTtsGeneratedAudio(audio);
- SherpaOnnxDestroyOfflineTts(tts);
- std::cout << "输入文本: " << text << std::endl;
- std::cout << "保存的文件: " << output_filename << std::endl;
- }
- int main(){
- // 语音识别 asr (sherpa-onnx-nemo-streaming-fast-conformer-transducer-en-80ms 模型)
- // 参考 decode-file-c-api.c
- // asr_1();
- // 语音识别 asr (sherpa-onnx-streaming-zipformer-ctc-multi-zh-hans-2023-12-13 模型)
- // 参考 streaming-ctc-buffered-tokens-c-api.c
- // asr_2();
- // 语音合成 tts
- // 参考 offline-tts-c-api.c
- tts();
- return 0;
- }
复制代码 3.java-api
和使用 c-api 一样,核心代码由 c++ 实现,java 只通过 jni 调用。所以只必要动态库和 java jni 的 jar 即可。
jni 库可以直接下载,也可以自己编译。预构建的 java jni 库下载地址,找对应版本的系统下载即可:
下载地址:
https://hf-mirror.com/csukuangfj/sherpa-onnx-libs/tree/main/jni
找一个版本然后将 so 库和 jar 一起下载下来:
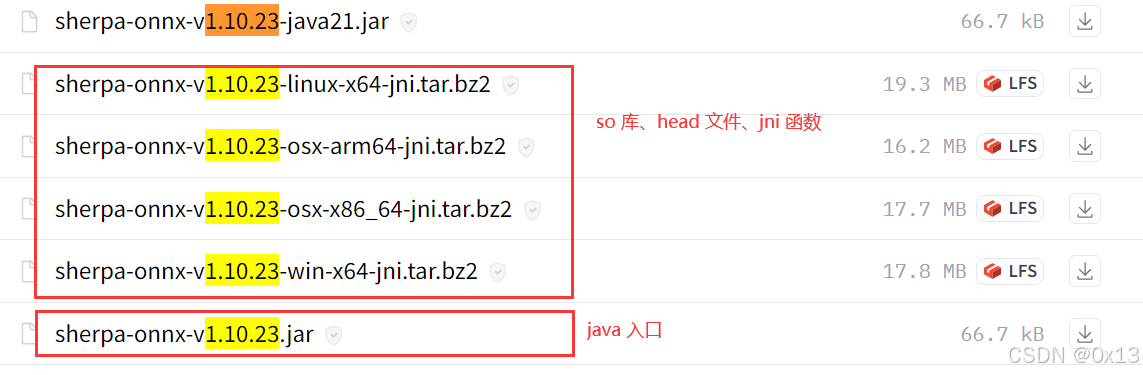
必要引入动态库和jar依赖:
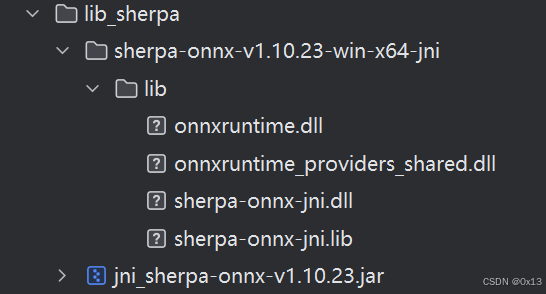
下面是参考源码写的测试用例:
- package tool.deeplearning;
- import com.k2fsa.sherpa.onnx.*;
- import java.io.File;
- /**
- * @desc : sherpa-onnx 的 asr(语音识别) + tts(语音合成) 推理
- * @auth : tyf
- * @date : 2024-10-16 10:51:14
- */
- public class sherpa_onnx {
- // 加载所有动态库
- public static void loadLib() throws Exception{
- String lib_path = new File("").getCanonicalPath()+ "\\lib_sherpa\\sherpa-onnx-v1.10.23-win-x64-jni\\lib\";
- String lib1 = lib_path + "onnxruntime.dll";
- String lib2 = lib_path + "onnxruntime_providers_shared.dll";
- String lib3 = lib_path + "sherpa-onnx-jni.dll";
- System.load(lib1);
- System.load(lib2);
- System.load(lib3);
- }
- // 语音识别 asr (sherpa-onnx-nemo-streaming-fast-conformer-transducer-en-80ms 模型)
- public static void asr_1(){
- String parent = "D:\\work\\workspace\\sherpa-onnx\\build\\sherpa-onnx-nemo-streaming-fast-conformer-transducer-en-80ms";
- String encoder = parent + "\\encoder.onnx";
- String decoder = parent + "\\decoder.onnx";
- String joiner = parent + "\\joiner.onnx";
- String tokens = parent + "\\tokens.txt";
- String waveFilename = parent + "\\test_wavs/0.wav";
- WaveReader reader = new WaveReader(waveFilename);
- OnlineTransducerModelConfig transducer = OnlineTransducerModelConfig.builder()
- .setEncoder(encoder)
- .setDecoder(decoder)
- .setJoiner(joiner)
- .build();
- OnlineModelConfig modelConfig = OnlineModelConfig.builder().setTransducer(transducer).setTokens(tokens).setNumThreads(1).setDebug(true).build();
- OnlineRecognizerConfig config =
- OnlineRecognizerConfig.builder()
- .setOnlineModelConfig(modelConfig)
- .setDecodingMethod("greedy_search")
- .build();
- OnlineRecognizer recognizer = new OnlineRecognizer(config);
- OnlineStream stream = recognizer.createStream();
- stream.acceptWaveform(reader.getSamples(), reader.getSampleRate());
- float[] tailPaddings = new float[(int) (0.8 * reader.getSampleRate())];
- stream.acceptWaveform(tailPaddings, reader.getSampleRate());
- while (recognizer.isReady(stream)) {
- recognizer.decode(stream);
- }
- String text = recognizer.getResult(stream).getText();
- System.out.printf("filename:%s\nresult:%s\n", waveFilename, text);
- stream.release();
- recognizer.release();
- }
- // 语音识别 asr (sherpa-onnx-streaming-zipformer-ctc-multi-zh-hans-2023-12-13 模型)
- public static void asr_2(){
- String parent = "D:\\work\\workspace\\sherpa-onnx\\build\\sherpa-onnx-streaming-zipformer-ctc-multi-zh-hans-2023-12-13\";
- String model = parent + "ctc-epoch-20-avg-1-chunk-16-left-128.onnx";
- String tokens = parent + "tokens.txt";
- String waveFilename = parent + "test_wavs\\DEV_T0000000000.wav";
- WaveReader reader = new WaveReader(waveFilename);
- OnlineZipformer2CtcModelConfig ctc = OnlineZipformer2CtcModelConfig.builder().setModel(model).build();
- OnlineModelConfig modelConfig = OnlineModelConfig.builder()
- .setZipformer2Ctc(ctc)
- .setTokens(tokens)
- .setNumThreads(1)
- .setDebug(true)
- .build();
- OnlineRecognizerConfig config = OnlineRecognizerConfig.builder()
- .setOnlineModelConfig(modelConfig)
- .setDecodingMethod("greedy_search")
- .build();
- OnlineRecognizer recognizer = new OnlineRecognizer(config);
- OnlineStream stream = recognizer.createStream();
- stream.acceptWaveform(reader.getSamples(), reader.getSampleRate());
- float[] tailPaddings = new float[(int) (0.3 * reader.getSampleRate())];
- stream.acceptWaveform(tailPaddings, reader.getSampleRate());
- while (recognizer.isReady(stream)) {
- recognizer.decode(stream);
- }
- String text = recognizer.getResult(stream).getText();
- System.out.printf("filename:%s\nresult:%s\n", waveFilename, text);
- stream.release();
- recognizer.release();
- }
- // 语音合成 tts (vits-melo-tts-zh_en 模型)
- public static void tts(){
- // please visit
- // https://github.com/k2-fsa/sherpa-onnx/releases/tag/tts-models
- // to download model files
- String parent = "D:\\work\\workspace\\sherpa-onnx\\build\\sherpa-onnx-vits-zh-ll\";
- String model = parent + "model.onnx";
- String tokens = parent + "tokens.txt";
- String lexicon = parent + "lexicon.txt";
- String dictDir = "dict";
- String ruleFsts =
- parent + "vits-zh-hf-fanchen-C/phone.fst,"+
- parent + "vits-zh-hf-fanchen-C/date.fst,"+
- parent + "vits-zh-hf-fanchen-C/number.fst";
- String text = "有问题,请拨打110或者手机18601239876。我们的价值观是真诚热爱!";
- OfflineTtsVitsModelConfig vitsModelConfig = OfflineTtsVitsModelConfig.builder()
- .setModel(model)
- .setTokens(tokens)
- .setLexicon(lexicon)
- .setDictDir(dictDir)
- .build();
- OfflineTtsModelConfig modelConfig = OfflineTtsModelConfig.builder()
- .setVits(vitsModelConfig)
- .setNumThreads(1)
- .setDebug(true)
- .build();
- OfflineTtsConfig config = OfflineTtsConfig.builder().setModel(modelConfig).setRuleFsts(ruleFsts).build();
- OfflineTts tts = new OfflineTts(config);
- int sid = 100;
- float speed = 1.0f;
- long start = System.currentTimeMillis();
- GeneratedAudio audio = tts.generate(text, sid, speed);
- long stop = System.currentTimeMillis();
- float timeElapsedSeconds = (stop - start) / 1000.0f;
- float audioDuration = audio.getSamples().length / (float) audio.getSampleRate();
- float real_time_factor = timeElapsedSeconds / audioDuration;
- String waveFilename = "tts-vits-zh.wav";
- audio.save(waveFilename);
- System.out.printf("-- elapsed : %.3f seconds\n", timeElapsedSeconds);
- System.out.printf("-- audio duration: %.3f seconds\n", timeElapsedSeconds);
- System.out.printf("-- real-time factor (RTF): %.3f\n", real_time_factor);
- System.out.printf("-- text: %s\n", text);
- System.out.printf("-- Saved to %s\n", waveFilename);
- tts.release();
- }
- public static void main(String[] args) throws Exception{
- // 加载动态库,注意 sherpa-onnx.jar 需要 jdk21
- loadLib();
- // 语音识别 asr (sherpa-onnx-nemo-streaming-fast-conformer-transducer-en-80ms 模型)
- // 参考 ./run-streaming-decode-file-transducer.sh 脚本及其 java 类
- asr_1();
- // 语音识别 asr (sherpa-onnx-streaming-zipformer-ctc-multi-zh-hans-2023-12-13 模型)
- // 参考 run-streaming-decode-file-ctc.sh 脚本及其 java 类
- asr_2();
- // 语音合成 tts (vits-melo-tts-zh_en 模型)
- tts();
- }
- }
复制代码 4.android 中使用
android 中使用就和 java-api 差不多了,编译 android 平台的动态库以及jni 的jar 包就可以使用了,直接用官方预构建的下载链接:
https://github.com/k2-fsa/sherpa-onnx/releases
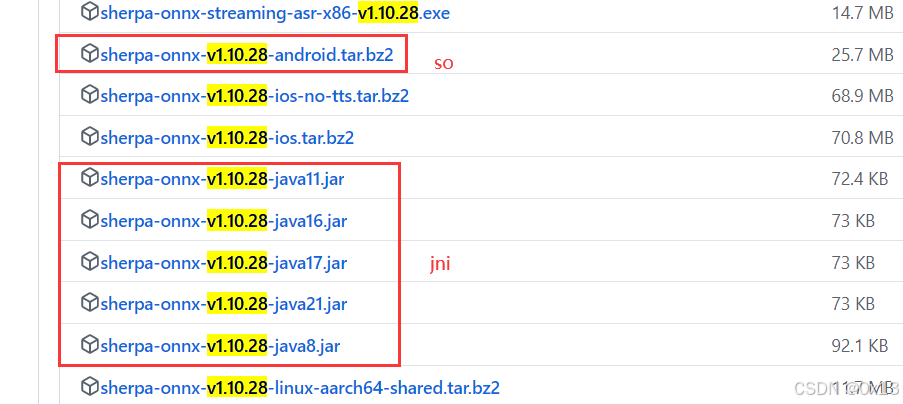
可以检察一下动态库符号(关键字sherpa ),对照 jar 中的 java api 举行调用即可:
nm -D libsherpa-onnx-jni.so | grep "sherpa"
在 android 中引入 so、jar:
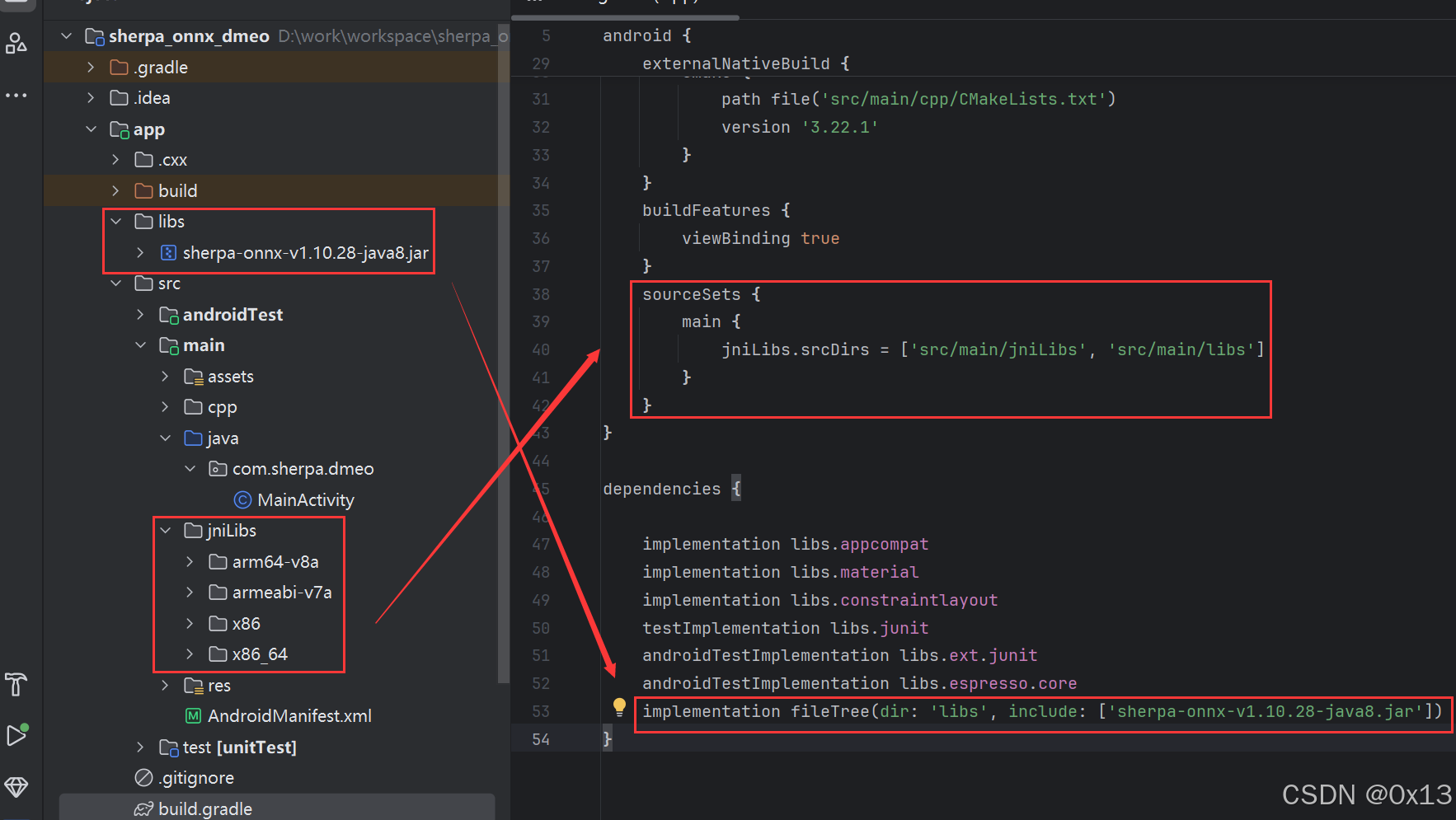
下面是参考实例的 kt 代码写的 java 测试 MainActivity:
- package com.sherpa.dmeo;
- import androidx.appcompat.app.AppCompatActivity;
- import android.os.Bundle;
- import android.util.Log;
- import com.k2fsa.sherpa.onnx.GeneratedAudio;
- import com.k2fsa.sherpa.onnx.OfflineTts;
- import com.k2fsa.sherpa.onnx.OfflineTtsConfig;
- import com.k2fsa.sherpa.onnx.OfflineTtsModelConfig;
- import com.k2fsa.sherpa.onnx.OfflineTtsVitsModelConfig;
- import com.sherpa.dmeo.databinding.ActivityMainBinding;
- import com.sherpa.dmeo.util.Tools;
- import java.io.File;
- import java.util.concurrent.Executors;
- /**
- * @desc : TTS/ASR 测试
- * @auth : tyf
- * @date : 2024-10-18 10:33:03
- */
- public class MainActivity extends AppCompatActivity {
- private ActivityMainBinding binding;
- private static String TAG = MainActivity.class.getName();
- @Override
- protected void onCreate(Bundle savedInstanceState) {
- super.onCreate(savedInstanceState);
- binding = ActivityMainBinding.inflate(getLayoutInflater());
- setContentView(binding.getRoot());
- // 语音合成测试
- Executors.newSingleThreadExecutor().submit(()->{
- // 递归复制模型文件到 app 存储路径
- Tools.setContext(this);
- Tools.copyAsset("vits-melo-tts-zh_en",Tools.path());
- String model = Tools.path() + "/vits-melo-tts-zh_en/model.onnx";
- String tokens = Tools.path() + "/vits-melo-tts-zh_en/tokens.txt";
- String lexicon = Tools.path() + "/vits-melo-tts-zh_en/lexicon.txt";
- String dictDir = Tools.path() + "/vits-melo-tts-zh_en/dict";
- String ruleFsts = Tools.path() + "/vits-melo-tts-zh_en/phone.fst," +
- Tools.path() + "/vits-melo-tts-zh_en/date.fst," +
- Tools.path() +"/vits-melo-tts-zh_en/number.fst," +
- Tools.path() +"/vits-melo-tts-zh_en/new_heteronym.fst";
- // 待生成文本
- String text = "在晨光初照的时分,\n" +
- "微风轻拂,花瓣轻舞,\n" +
- "小溪潺潺,诉说心事,\n" +
- "阳光透过树梢,洒下温暖。\n" +
- "\n" +
- "远山如黛,静默守望,\n" +
- "白云悠悠,似梦似幻,\n" +
- "时光流转,岁月如歌,\n" +
- "愿心中永存这份宁静。\n" +
- "\n" +
- "无论何时,心怀希望,\n" +
- "在每一个晨曦中起舞,\n" +
- "追逐梦想,勇往直前,\n" +
- "让生命绽放出灿烂的光彩。";
- // 输出wav文件
- String waveFilename = Tools.path() + "/tts-vits-zh.wav";
- Log.d(TAG,"开始语音合成!");
- OfflineTtsVitsModelConfig vitsModelConfig = OfflineTtsVitsModelConfig.builder()
- .setModel(model)
- .setTokens(tokens)
- .setLexicon(lexicon)
- .setDictDir(dictDir)
- .build();
- OfflineTtsModelConfig modelConfig = OfflineTtsModelConfig.builder()
- .setVits(vitsModelConfig)
- .setNumThreads(1)
- .setDebug(true)
- .build();
- OfflineTtsConfig config = OfflineTtsConfig.builder().setModel(modelConfig).setRuleFsts(ruleFsts).build();
- OfflineTts tts = new OfflineTts(config);
- // 语速和说话人
- int sid = 100;
- float speed = 1.0f;
- long start = System.currentTimeMillis();
- GeneratedAudio audio = tts.generate(text, sid, speed);
- long stop = System.currentTimeMillis();
- float timeElapsedSeconds = (stop - start) / 1000.0f;
- float audioDuration = audio.getSamples().length / (float) audio.getSampleRate();
- float real_time_factor = timeElapsedSeconds / audioDuration;
- audio.save(waveFilename);
- Log.d(TAG, String.format("-- elapsed : %.3f seconds", timeElapsedSeconds));
- Log.d(TAG, String.format("-- audio duration: %.3f seconds", timeElapsedSeconds));
- Log.d(TAG, String.format("-- real-time factor (RTF): %.3f", real_time_factor));
- Log.d(TAG, String.format("-- text: %s", text));
- Log.d(TAG, String.format("-- Saved to %s", waveFilename));
- Log.d(TAG,"音频合成:"+waveFilename+",是否成功:"+new File(waveFilename).exists());
- tts.release();
- // 播放 wav
- Tools.play(waveFilename);
- });
- }
- }
复制代码 android 项目示例代码:
https://github.com/TangYuFan/deeplearn-mobile/tree/main/android_sherpa_onnx_ars_dmeo
https://github.com/TangYuFan/deeplearn-mobile/tree/main/android_sherpa_onnx_tts_dmeo
免责声明:如果侵犯了您的权益,请联系站长,我们会及时删除侵权内容,谢谢合作!更多信息从访问主页:qidao123.com:ToB企服之家,中国第一个企服评测及商务社交产业平台。 |